Averaging Bayesian posteriors [duplicate]
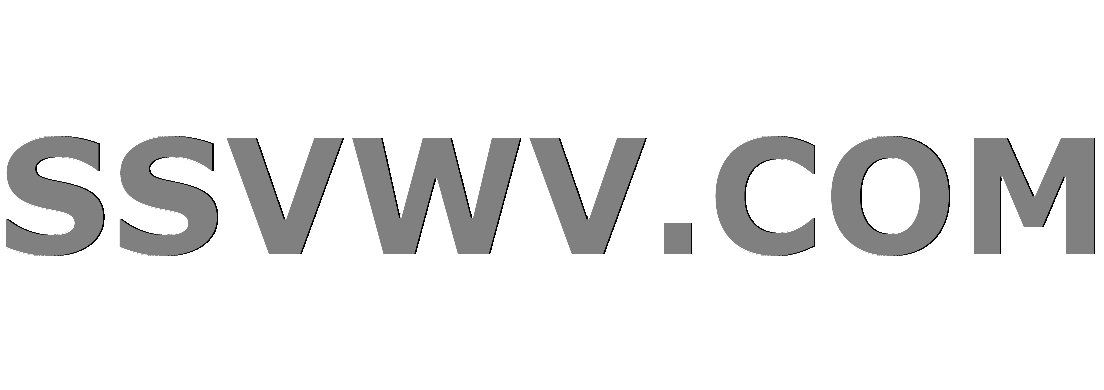
Multi tool use
up vote
4
down vote
favorite
This question already has an answer here:
If N Bayesians estimate the probability of something, how do you aggregate their estimations?
1 answer
Does it make sense to use different uninformative priors and then take the mean of all the posteriors? For example, use (0,0), (0.5, 0.5), (1, 1) and calculate three posteriors and then take the mean. My thinking is that this will minimize the error from using the “wrong” prior (so to speak). This is of course being done, if we cannot have subjective priors. Has this been looked at?
bayesian
New contributor
Humean David is a new contributor to this site. Take care in asking for clarification, commenting, and answering.
Check out our Code of Conduct.
marked as duplicate by Ben, kjetil b halvorsen, Carl, mkt, Peter Flom♦ Dec 7 at 12:36
This question has been asked before and already has an answer. If those answers do not fully address your question, please ask a new question.
|
show 3 more comments
up vote
4
down vote
favorite
This question already has an answer here:
If N Bayesians estimate the probability of something, how do you aggregate their estimations?
1 answer
Does it make sense to use different uninformative priors and then take the mean of all the posteriors? For example, use (0,0), (0.5, 0.5), (1, 1) and calculate three posteriors and then take the mean. My thinking is that this will minimize the error from using the “wrong” prior (so to speak). This is of course being done, if we cannot have subjective priors. Has this been looked at?
bayesian
New contributor
Humean David is a new contributor to this site. Take care in asking for clarification, commenting, and answering.
Check out our Code of Conduct.
marked as duplicate by Ben, kjetil b halvorsen, Carl, mkt, Peter Flom♦ Dec 7 at 12:36
This question has been asked before and already has an answer. If those answers do not fully address your question, please ask a new question.
4
If you cannot pick the priors, why not using hyperpriors for the parameter of the priors? This is what is usually done in Bayesian setting.
– Tim♦
Dec 6 at 12:50
Hi Tim, thank you. Can you please expand? What would be the hyperprior for, let’s say uniform prior (1, 1)?
– Humean David
Dec 6 at 13:31
1
Averaging what? If you average returned probabilities, they may not sum/integrate to one any more. Why averaging three arbitrary priors would solve anything? Why not two, or 62 different priors? Using hyperpriors does exactly that: it lets you integrate over a whole range of possible priors.
– Tim♦
Dec 6 at 14:51
1
Since, as I said, using hyperpriors seem to do exactly the same thing but using method that (a) is pretty standard in Bayesian setting, (b) has theoretical justification within the Bayesian framework, so why instead would you like to use arbitrary method, that potentially gives you uninterpretable results (probabilities that do not sum to one)?
– Tim♦
Dec 6 at 15:49
1
Is OP referring to model averaging? Model averaging is not motivated by uncertainty in parameters for priors. Like @Tim said, use a hyperprior. It is motivated by the uncertainty inherent to any modeling exercise. You can use something like the WAIC to create weights for each candidate model, then compute weighted averages.
– Heteroskedastic Jim
Dec 6 at 17:21
|
show 3 more comments
up vote
4
down vote
favorite
up vote
4
down vote
favorite
This question already has an answer here:
If N Bayesians estimate the probability of something, how do you aggregate their estimations?
1 answer
Does it make sense to use different uninformative priors and then take the mean of all the posteriors? For example, use (0,0), (0.5, 0.5), (1, 1) and calculate three posteriors and then take the mean. My thinking is that this will minimize the error from using the “wrong” prior (so to speak). This is of course being done, if we cannot have subjective priors. Has this been looked at?
bayesian
New contributor
Humean David is a new contributor to this site. Take care in asking for clarification, commenting, and answering.
Check out our Code of Conduct.
This question already has an answer here:
If N Bayesians estimate the probability of something, how do you aggregate their estimations?
1 answer
Does it make sense to use different uninformative priors and then take the mean of all the posteriors? For example, use (0,0), (0.5, 0.5), (1, 1) and calculate three posteriors and then take the mean. My thinking is that this will minimize the error from using the “wrong” prior (so to speak). This is of course being done, if we cannot have subjective priors. Has this been looked at?
This question already has an answer here:
If N Bayesians estimate the probability of something, how do you aggregate their estimations?
1 answer
bayesian
bayesian
New contributor
Humean David is a new contributor to this site. Take care in asking for clarification, commenting, and answering.
Check out our Code of Conduct.
New contributor
Humean David is a new contributor to this site. Take care in asking for clarification, commenting, and answering.
Check out our Code of Conduct.
New contributor
Humean David is a new contributor to this site. Take care in asking for clarification, commenting, and answering.
Check out our Code of Conduct.
asked Dec 6 at 12:44


Humean David
212
212
New contributor
Humean David is a new contributor to this site. Take care in asking for clarification, commenting, and answering.
Check out our Code of Conduct.
New contributor
Humean David is a new contributor to this site. Take care in asking for clarification, commenting, and answering.
Check out our Code of Conduct.
Humean David is a new contributor to this site. Take care in asking for clarification, commenting, and answering.
Check out our Code of Conduct.
marked as duplicate by Ben, kjetil b halvorsen, Carl, mkt, Peter Flom♦ Dec 7 at 12:36
This question has been asked before and already has an answer. If those answers do not fully address your question, please ask a new question.
marked as duplicate by Ben, kjetil b halvorsen, Carl, mkt, Peter Flom♦ Dec 7 at 12:36
This question has been asked before and already has an answer. If those answers do not fully address your question, please ask a new question.
4
If you cannot pick the priors, why not using hyperpriors for the parameter of the priors? This is what is usually done in Bayesian setting.
– Tim♦
Dec 6 at 12:50
Hi Tim, thank you. Can you please expand? What would be the hyperprior for, let’s say uniform prior (1, 1)?
– Humean David
Dec 6 at 13:31
1
Averaging what? If you average returned probabilities, they may not sum/integrate to one any more. Why averaging three arbitrary priors would solve anything? Why not two, or 62 different priors? Using hyperpriors does exactly that: it lets you integrate over a whole range of possible priors.
– Tim♦
Dec 6 at 14:51
1
Since, as I said, using hyperpriors seem to do exactly the same thing but using method that (a) is pretty standard in Bayesian setting, (b) has theoretical justification within the Bayesian framework, so why instead would you like to use arbitrary method, that potentially gives you uninterpretable results (probabilities that do not sum to one)?
– Tim♦
Dec 6 at 15:49
1
Is OP referring to model averaging? Model averaging is not motivated by uncertainty in parameters for priors. Like @Tim said, use a hyperprior. It is motivated by the uncertainty inherent to any modeling exercise. You can use something like the WAIC to create weights for each candidate model, then compute weighted averages.
– Heteroskedastic Jim
Dec 6 at 17:21
|
show 3 more comments
4
If you cannot pick the priors, why not using hyperpriors for the parameter of the priors? This is what is usually done in Bayesian setting.
– Tim♦
Dec 6 at 12:50
Hi Tim, thank you. Can you please expand? What would be the hyperprior for, let’s say uniform prior (1, 1)?
– Humean David
Dec 6 at 13:31
1
Averaging what? If you average returned probabilities, they may not sum/integrate to one any more. Why averaging three arbitrary priors would solve anything? Why not two, or 62 different priors? Using hyperpriors does exactly that: it lets you integrate over a whole range of possible priors.
– Tim♦
Dec 6 at 14:51
1
Since, as I said, using hyperpriors seem to do exactly the same thing but using method that (a) is pretty standard in Bayesian setting, (b) has theoretical justification within the Bayesian framework, so why instead would you like to use arbitrary method, that potentially gives you uninterpretable results (probabilities that do not sum to one)?
– Tim♦
Dec 6 at 15:49
1
Is OP referring to model averaging? Model averaging is not motivated by uncertainty in parameters for priors. Like @Tim said, use a hyperprior. It is motivated by the uncertainty inherent to any modeling exercise. You can use something like the WAIC to create weights for each candidate model, then compute weighted averages.
– Heteroskedastic Jim
Dec 6 at 17:21
4
4
If you cannot pick the priors, why not using hyperpriors for the parameter of the priors? This is what is usually done in Bayesian setting.
– Tim♦
Dec 6 at 12:50
If you cannot pick the priors, why not using hyperpriors for the parameter of the priors? This is what is usually done in Bayesian setting.
– Tim♦
Dec 6 at 12:50
Hi Tim, thank you. Can you please expand? What would be the hyperprior for, let’s say uniform prior (1, 1)?
– Humean David
Dec 6 at 13:31
Hi Tim, thank you. Can you please expand? What would be the hyperprior for, let’s say uniform prior (1, 1)?
– Humean David
Dec 6 at 13:31
1
1
Averaging what? If you average returned probabilities, they may not sum/integrate to one any more. Why averaging three arbitrary priors would solve anything? Why not two, or 62 different priors? Using hyperpriors does exactly that: it lets you integrate over a whole range of possible priors.
– Tim♦
Dec 6 at 14:51
Averaging what? If you average returned probabilities, they may not sum/integrate to one any more. Why averaging three arbitrary priors would solve anything? Why not two, or 62 different priors? Using hyperpriors does exactly that: it lets you integrate over a whole range of possible priors.
– Tim♦
Dec 6 at 14:51
1
1
Since, as I said, using hyperpriors seem to do exactly the same thing but using method that (a) is pretty standard in Bayesian setting, (b) has theoretical justification within the Bayesian framework, so why instead would you like to use arbitrary method, that potentially gives you uninterpretable results (probabilities that do not sum to one)?
– Tim♦
Dec 6 at 15:49
Since, as I said, using hyperpriors seem to do exactly the same thing but using method that (a) is pretty standard in Bayesian setting, (b) has theoretical justification within the Bayesian framework, so why instead would you like to use arbitrary method, that potentially gives you uninterpretable results (probabilities that do not sum to one)?
– Tim♦
Dec 6 at 15:49
1
1
Is OP referring to model averaging? Model averaging is not motivated by uncertainty in parameters for priors. Like @Tim said, use a hyperprior. It is motivated by the uncertainty inherent to any modeling exercise. You can use something like the WAIC to create weights for each candidate model, then compute weighted averages.
– Heteroskedastic Jim
Dec 6 at 17:21
Is OP referring to model averaging? Model averaging is not motivated by uncertainty in parameters for priors. Like @Tim said, use a hyperprior. It is motivated by the uncertainty inherent to any modeling exercise. You can use something like the WAIC to create weights for each candidate model, then compute weighted averages.
– Heteroskedastic Jim
Dec 6 at 17:21
|
show 3 more comments
3 Answers
3
active
oldest
votes
up vote
7
down vote
No, please don't do that. The strength of Bayesian solutions is that they are subjective. The prior is a good thing and not something to be avoided. What you are doing is assuming the sample you are working with is a good sample, and your personal knowledge is of no value. It appears your fear is that of introducing bias.
All Bayesian methods are biased. That is not a bad thing. It is just a fact. As the sample size becomes very large, the Bayesian models become just barely biased if that helps.
So let us break apart some assumptions in your posting that may or may not have the results you intended. I am going to work back to front. You want to know about averaging posterior means.
The posterior mean minimizes quadratic loss. Unlike a Frequentist solution where this is the answer, this is not automatically true for Bayesian thought. To give you an example of where a posterior or a Frequentist mean would be a catastrophically bad idea consider the following case.
You have an underwater engineering project where you have to pour, in a single pour, a concrete structure. If you pour too little, you will have to destroy the object and start over. If you end up with too much concrete, then you just discard the excess and never pour it. Just to make this interesting, let us make this an expensive event. Each cubic foot costs $1,000, and the true parameter is 1,000 cubic feet. If you purchase and pour 999 cubic feet, you have to destroy it, buy new concrete and start over. The cost is $999,000 in lost concrete plus demolition. If you purchase 1,001 cubic feet, your waste is $1,000.
If you choose quadratic loss to estimate the population parameter, half the time you will take catastrophic losses, if you use the true cost function created by errors, you can construct a weighted posterior point estimator that minimizes the average risk of loss.
There is nothing magic about the posterior mean. It should be used when your real loss from being wrong is quadratic. A quadratic loss is quite common in the real world, particularly in gambling. Remember this is not the loss created by gambling wrong, but by estimating the parameter wrong.
Is your real-world loss quadratic, if yes, then use the posterior mean, but if no then use the real loss function?
Bayesian solutions proper create an entire posterior density. If you are going to choose a point from it make sure your point makes sense to you. As a rule of thumb, you cannot average them as many do not have good compositional properties.
Now to the prior. Tim mentioned using hyperparameters as your alternative. That is taking the two parameters for the beta prior and creating functions to cover prior parameter uncertainty. It is a sort of prior averaging. Before you go there, you should think about what information you have. If you do not, then the good intrinsic properties of Bayesian methods go away, and the bias does become a problem.
Let us start with the Beta (0,0) prior. It has infinite mass at 0 and 1 and minimal mass at 50%. Do you really believe it is almost certainly exactly zero or one? Do you really believe it is unlikely to be one half? Make a picture of it; does it match your beliefs? The Beta (.5,.5), Jeffreys prior, does the same thing. The derivative is different, but there is still infinite weight on zero and one. Because they are symmetric priors, they also bias the expectation toward one half. Do you believe the true value of the parameter to have a fifty percent chance of being on either side of the one-half mark?
The Haldane prior was created for a real-world problem, the Beta (0,0). It is often the case in chemistry that you have to destroy your sample and you may always have a sample size of one. Is it soluble in water or is it not soluble in water (0-1)? Did it dissolve? Now you can make a proper statistical statement about it as you do have a prior. It is also the prior that maps to the Frequentist solution for the binomial. It is uninformative, but as you can see, you have three "uninformative priors." All priors convey information, even uninformative ones.
The Jeffreys prior was created to be invariant to monotonic transformations. That matters because probability statements can move with transformation. Only the maximum likelihood estimator is automatically invariant under transformation. It has more information in it than the Haldane prior and does not allow double-sided coins, which the Haldane prior does. Its virtue is that you can make transformations of the data without a consequence to your inference.
Finally, there is the uniform distribution, the Beta (1,1). Except for the case of a double-headed coin, it weights all possibilities equally.
Now here is the rub, do you really have no out-of-sample information about the true value of the parameter? For example, if you were doing bankruptcy research, you could estimate bankruptcy rates from loan interest rates. If bankruptcy rates are high, then loan rates would have to be higher to cover the risk and make a profit. Minimally, success is more likely than failure for seasoned businesses at least. So, knowing nothing else, you should have a Beta(1,2), the triangular distribution, $pi(theta)=1-theta$.
Now let us imagine you speak to a friend that is a bank loan officer and ask about loan losses and you are told it is 2%. Of course, that includes losses other than bankruptcy. Now you have a Beta(2,98), so $pi(theta)=9702theta(1-theta)^{97}$.
That is a lot of information, and you biased your outcome. That is a good thing because the bias isn't pure bias. It is information plus noise. It is the noise that you do not want, but cannot avoid. Look at the graph for this prior.
It is giving material weight to anything less than eight percent. It has reduced your search space and it has regularized the long run rate so that if you hit a run, such as bankruptcies in a major recession, it is normalized so that it will be perceived by the math as unlikely (forgive the anthropomorphization) and be underweighted.
More importantly, you have used professional information from outside your sample. That is perfectly good information. It is biased in that it is one bank's experience and you could certainly construct a better prior if you got more information and took more time to look.
Just as important, you preserved two properties that the Bayesian bias pays for, admissibility and coherence.
If you use your true prior then Bayesian methods cannot be stochastically dominated. Further, if you use your true prior then you can place fair bets and function as a bookie. You may not think of that as valuable, but a grocer is a bookie for fruit. They are gambling on inventory and placing a bet on how much you will buy and at what price. Frequentist methods are not coherent.
Now lets talk about the bad side of this prior, the one that worries you. Let us assume you collect a sample of 10,000 firms and over the period of time 10 go bankrupt. The sample bankruptcy rate is .1% but the posterior mean is .11%. Ha, you say, there is your bias. That is not the bias. The bias is from the true rate. If the true rate is .12% then it is biased downward. If it is .09% then it is biased upward.
You do not want to worry about the bias or getting the wrong prior. What you want to do is find the prior that works best for your problem, that is to say, it encodes your knowledge, whatever it may be, as closely as you can make a formula do that.
If you want an unbiased estimator do not use Bayesian methods. If you want to get an accurate estimator, encode your knowledge into the prior. If you have several possible priors, multiply them together and normalize them to one or use hyperparameters.
Part of your prior will be inaccurate just as a sample contains outliers or non-standardized components. Samples are bad too, you usually just cannot see it because you do not look. The prior regularizes and normalizes your sample.
1
If I could give you 100 upvotes, I would. Thank you for the detailed answer. You answered all my questions. Thank you Kindly!!!
– Humean David
Dec 6 at 17:26
@HumeanDavid, then you should also accept his answer :)
– Avraham
Dec 6 at 22:55
add a comment |
up vote
7
down vote
The fundamental difficulty with averaging the posteriors is that the data has no say in the weight of the said posteriors. If the global prior is the average of three possible priors (with weights $1/3$, $1/3$, $1/3$), the posterior will not be the average of the corresponding posteriors with weights $1/3$, $1/3$, $1/3$, as the weights will depend on the data.
Check out the keyword Bayesian model averaging
for more details. As for instance in Hoeting et al. (1999). Or these slides.
1
It’s worth mentioning that, just because naive averaging disagrees with BMA, this does not mean naive averaging will actually perform worse in practice. For example, my experience is that Bayesian CART often performs worse than naively sampling decision trees from the prior and averaging together the associated predictions. This is in terms of prediction error on heldout data, say.
– guy
Dec 6 at 22:56
add a comment |
up vote
1
down vote
Assume your posterior is a multimodal distribution. If your different priors are chosen such that you only get one region of high probability per prior, then your average of the means would lie somewhere between these means, but in probably in a region with low mass.
So better not taking the average of means.
New contributor
Balou is a new contributor to this site. Take care in asking for clarification, commenting, and answering.
Check out our Code of Conduct.
add a comment |
3 Answers
3
active
oldest
votes
3 Answers
3
active
oldest
votes
active
oldest
votes
active
oldest
votes
up vote
7
down vote
No, please don't do that. The strength of Bayesian solutions is that they are subjective. The prior is a good thing and not something to be avoided. What you are doing is assuming the sample you are working with is a good sample, and your personal knowledge is of no value. It appears your fear is that of introducing bias.
All Bayesian methods are biased. That is not a bad thing. It is just a fact. As the sample size becomes very large, the Bayesian models become just barely biased if that helps.
So let us break apart some assumptions in your posting that may or may not have the results you intended. I am going to work back to front. You want to know about averaging posterior means.
The posterior mean minimizes quadratic loss. Unlike a Frequentist solution where this is the answer, this is not automatically true for Bayesian thought. To give you an example of where a posterior or a Frequentist mean would be a catastrophically bad idea consider the following case.
You have an underwater engineering project where you have to pour, in a single pour, a concrete structure. If you pour too little, you will have to destroy the object and start over. If you end up with too much concrete, then you just discard the excess and never pour it. Just to make this interesting, let us make this an expensive event. Each cubic foot costs $1,000, and the true parameter is 1,000 cubic feet. If you purchase and pour 999 cubic feet, you have to destroy it, buy new concrete and start over. The cost is $999,000 in lost concrete plus demolition. If you purchase 1,001 cubic feet, your waste is $1,000.
If you choose quadratic loss to estimate the population parameter, half the time you will take catastrophic losses, if you use the true cost function created by errors, you can construct a weighted posterior point estimator that minimizes the average risk of loss.
There is nothing magic about the posterior mean. It should be used when your real loss from being wrong is quadratic. A quadratic loss is quite common in the real world, particularly in gambling. Remember this is not the loss created by gambling wrong, but by estimating the parameter wrong.
Is your real-world loss quadratic, if yes, then use the posterior mean, but if no then use the real loss function?
Bayesian solutions proper create an entire posterior density. If you are going to choose a point from it make sure your point makes sense to you. As a rule of thumb, you cannot average them as many do not have good compositional properties.
Now to the prior. Tim mentioned using hyperparameters as your alternative. That is taking the two parameters for the beta prior and creating functions to cover prior parameter uncertainty. It is a sort of prior averaging. Before you go there, you should think about what information you have. If you do not, then the good intrinsic properties of Bayesian methods go away, and the bias does become a problem.
Let us start with the Beta (0,0) prior. It has infinite mass at 0 and 1 and minimal mass at 50%. Do you really believe it is almost certainly exactly zero or one? Do you really believe it is unlikely to be one half? Make a picture of it; does it match your beliefs? The Beta (.5,.5), Jeffreys prior, does the same thing. The derivative is different, but there is still infinite weight on zero and one. Because they are symmetric priors, they also bias the expectation toward one half. Do you believe the true value of the parameter to have a fifty percent chance of being on either side of the one-half mark?
The Haldane prior was created for a real-world problem, the Beta (0,0). It is often the case in chemistry that you have to destroy your sample and you may always have a sample size of one. Is it soluble in water or is it not soluble in water (0-1)? Did it dissolve? Now you can make a proper statistical statement about it as you do have a prior. It is also the prior that maps to the Frequentist solution for the binomial. It is uninformative, but as you can see, you have three "uninformative priors." All priors convey information, even uninformative ones.
The Jeffreys prior was created to be invariant to monotonic transformations. That matters because probability statements can move with transformation. Only the maximum likelihood estimator is automatically invariant under transformation. It has more information in it than the Haldane prior and does not allow double-sided coins, which the Haldane prior does. Its virtue is that you can make transformations of the data without a consequence to your inference.
Finally, there is the uniform distribution, the Beta (1,1). Except for the case of a double-headed coin, it weights all possibilities equally.
Now here is the rub, do you really have no out-of-sample information about the true value of the parameter? For example, if you were doing bankruptcy research, you could estimate bankruptcy rates from loan interest rates. If bankruptcy rates are high, then loan rates would have to be higher to cover the risk and make a profit. Minimally, success is more likely than failure for seasoned businesses at least. So, knowing nothing else, you should have a Beta(1,2), the triangular distribution, $pi(theta)=1-theta$.
Now let us imagine you speak to a friend that is a bank loan officer and ask about loan losses and you are told it is 2%. Of course, that includes losses other than bankruptcy. Now you have a Beta(2,98), so $pi(theta)=9702theta(1-theta)^{97}$.
That is a lot of information, and you biased your outcome. That is a good thing because the bias isn't pure bias. It is information plus noise. It is the noise that you do not want, but cannot avoid. Look at the graph for this prior.
It is giving material weight to anything less than eight percent. It has reduced your search space and it has regularized the long run rate so that if you hit a run, such as bankruptcies in a major recession, it is normalized so that it will be perceived by the math as unlikely (forgive the anthropomorphization) and be underweighted.
More importantly, you have used professional information from outside your sample. That is perfectly good information. It is biased in that it is one bank's experience and you could certainly construct a better prior if you got more information and took more time to look.
Just as important, you preserved two properties that the Bayesian bias pays for, admissibility and coherence.
If you use your true prior then Bayesian methods cannot be stochastically dominated. Further, if you use your true prior then you can place fair bets and function as a bookie. You may not think of that as valuable, but a grocer is a bookie for fruit. They are gambling on inventory and placing a bet on how much you will buy and at what price. Frequentist methods are not coherent.
Now lets talk about the bad side of this prior, the one that worries you. Let us assume you collect a sample of 10,000 firms and over the period of time 10 go bankrupt. The sample bankruptcy rate is .1% but the posterior mean is .11%. Ha, you say, there is your bias. That is not the bias. The bias is from the true rate. If the true rate is .12% then it is biased downward. If it is .09% then it is biased upward.
You do not want to worry about the bias or getting the wrong prior. What you want to do is find the prior that works best for your problem, that is to say, it encodes your knowledge, whatever it may be, as closely as you can make a formula do that.
If you want an unbiased estimator do not use Bayesian methods. If you want to get an accurate estimator, encode your knowledge into the prior. If you have several possible priors, multiply them together and normalize them to one or use hyperparameters.
Part of your prior will be inaccurate just as a sample contains outliers or non-standardized components. Samples are bad too, you usually just cannot see it because you do not look. The prior regularizes and normalizes your sample.
1
If I could give you 100 upvotes, I would. Thank you for the detailed answer. You answered all my questions. Thank you Kindly!!!
– Humean David
Dec 6 at 17:26
@HumeanDavid, then you should also accept his answer :)
– Avraham
Dec 6 at 22:55
add a comment |
up vote
7
down vote
No, please don't do that. The strength of Bayesian solutions is that they are subjective. The prior is a good thing and not something to be avoided. What you are doing is assuming the sample you are working with is a good sample, and your personal knowledge is of no value. It appears your fear is that of introducing bias.
All Bayesian methods are biased. That is not a bad thing. It is just a fact. As the sample size becomes very large, the Bayesian models become just barely biased if that helps.
So let us break apart some assumptions in your posting that may or may not have the results you intended. I am going to work back to front. You want to know about averaging posterior means.
The posterior mean minimizes quadratic loss. Unlike a Frequentist solution where this is the answer, this is not automatically true for Bayesian thought. To give you an example of where a posterior or a Frequentist mean would be a catastrophically bad idea consider the following case.
You have an underwater engineering project where you have to pour, in a single pour, a concrete structure. If you pour too little, you will have to destroy the object and start over. If you end up with too much concrete, then you just discard the excess and never pour it. Just to make this interesting, let us make this an expensive event. Each cubic foot costs $1,000, and the true parameter is 1,000 cubic feet. If you purchase and pour 999 cubic feet, you have to destroy it, buy new concrete and start over. The cost is $999,000 in lost concrete plus demolition. If you purchase 1,001 cubic feet, your waste is $1,000.
If you choose quadratic loss to estimate the population parameter, half the time you will take catastrophic losses, if you use the true cost function created by errors, you can construct a weighted posterior point estimator that minimizes the average risk of loss.
There is nothing magic about the posterior mean. It should be used when your real loss from being wrong is quadratic. A quadratic loss is quite common in the real world, particularly in gambling. Remember this is not the loss created by gambling wrong, but by estimating the parameter wrong.
Is your real-world loss quadratic, if yes, then use the posterior mean, but if no then use the real loss function?
Bayesian solutions proper create an entire posterior density. If you are going to choose a point from it make sure your point makes sense to you. As a rule of thumb, you cannot average them as many do not have good compositional properties.
Now to the prior. Tim mentioned using hyperparameters as your alternative. That is taking the two parameters for the beta prior and creating functions to cover prior parameter uncertainty. It is a sort of prior averaging. Before you go there, you should think about what information you have. If you do not, then the good intrinsic properties of Bayesian methods go away, and the bias does become a problem.
Let us start with the Beta (0,0) prior. It has infinite mass at 0 and 1 and minimal mass at 50%. Do you really believe it is almost certainly exactly zero or one? Do you really believe it is unlikely to be one half? Make a picture of it; does it match your beliefs? The Beta (.5,.5), Jeffreys prior, does the same thing. The derivative is different, but there is still infinite weight on zero and one. Because they are symmetric priors, they also bias the expectation toward one half. Do you believe the true value of the parameter to have a fifty percent chance of being on either side of the one-half mark?
The Haldane prior was created for a real-world problem, the Beta (0,0). It is often the case in chemistry that you have to destroy your sample and you may always have a sample size of one. Is it soluble in water or is it not soluble in water (0-1)? Did it dissolve? Now you can make a proper statistical statement about it as you do have a prior. It is also the prior that maps to the Frequentist solution for the binomial. It is uninformative, but as you can see, you have three "uninformative priors." All priors convey information, even uninformative ones.
The Jeffreys prior was created to be invariant to monotonic transformations. That matters because probability statements can move with transformation. Only the maximum likelihood estimator is automatically invariant under transformation. It has more information in it than the Haldane prior and does not allow double-sided coins, which the Haldane prior does. Its virtue is that you can make transformations of the data without a consequence to your inference.
Finally, there is the uniform distribution, the Beta (1,1). Except for the case of a double-headed coin, it weights all possibilities equally.
Now here is the rub, do you really have no out-of-sample information about the true value of the parameter? For example, if you were doing bankruptcy research, you could estimate bankruptcy rates from loan interest rates. If bankruptcy rates are high, then loan rates would have to be higher to cover the risk and make a profit. Minimally, success is more likely than failure for seasoned businesses at least. So, knowing nothing else, you should have a Beta(1,2), the triangular distribution, $pi(theta)=1-theta$.
Now let us imagine you speak to a friend that is a bank loan officer and ask about loan losses and you are told it is 2%. Of course, that includes losses other than bankruptcy. Now you have a Beta(2,98), so $pi(theta)=9702theta(1-theta)^{97}$.
That is a lot of information, and you biased your outcome. That is a good thing because the bias isn't pure bias. It is information plus noise. It is the noise that you do not want, but cannot avoid. Look at the graph for this prior.
It is giving material weight to anything less than eight percent. It has reduced your search space and it has regularized the long run rate so that if you hit a run, such as bankruptcies in a major recession, it is normalized so that it will be perceived by the math as unlikely (forgive the anthropomorphization) and be underweighted.
More importantly, you have used professional information from outside your sample. That is perfectly good information. It is biased in that it is one bank's experience and you could certainly construct a better prior if you got more information and took more time to look.
Just as important, you preserved two properties that the Bayesian bias pays for, admissibility and coherence.
If you use your true prior then Bayesian methods cannot be stochastically dominated. Further, if you use your true prior then you can place fair bets and function as a bookie. You may not think of that as valuable, but a grocer is a bookie for fruit. They are gambling on inventory and placing a bet on how much you will buy and at what price. Frequentist methods are not coherent.
Now lets talk about the bad side of this prior, the one that worries you. Let us assume you collect a sample of 10,000 firms and over the period of time 10 go bankrupt. The sample bankruptcy rate is .1% but the posterior mean is .11%. Ha, you say, there is your bias. That is not the bias. The bias is from the true rate. If the true rate is .12% then it is biased downward. If it is .09% then it is biased upward.
You do not want to worry about the bias or getting the wrong prior. What you want to do is find the prior that works best for your problem, that is to say, it encodes your knowledge, whatever it may be, as closely as you can make a formula do that.
If you want an unbiased estimator do not use Bayesian methods. If you want to get an accurate estimator, encode your knowledge into the prior. If you have several possible priors, multiply them together and normalize them to one or use hyperparameters.
Part of your prior will be inaccurate just as a sample contains outliers or non-standardized components. Samples are bad too, you usually just cannot see it because you do not look. The prior regularizes and normalizes your sample.
1
If I could give you 100 upvotes, I would. Thank you for the detailed answer. You answered all my questions. Thank you Kindly!!!
– Humean David
Dec 6 at 17:26
@HumeanDavid, then you should also accept his answer :)
– Avraham
Dec 6 at 22:55
add a comment |
up vote
7
down vote
up vote
7
down vote
No, please don't do that. The strength of Bayesian solutions is that they are subjective. The prior is a good thing and not something to be avoided. What you are doing is assuming the sample you are working with is a good sample, and your personal knowledge is of no value. It appears your fear is that of introducing bias.
All Bayesian methods are biased. That is not a bad thing. It is just a fact. As the sample size becomes very large, the Bayesian models become just barely biased if that helps.
So let us break apart some assumptions in your posting that may or may not have the results you intended. I am going to work back to front. You want to know about averaging posterior means.
The posterior mean minimizes quadratic loss. Unlike a Frequentist solution where this is the answer, this is not automatically true for Bayesian thought. To give you an example of where a posterior or a Frequentist mean would be a catastrophically bad idea consider the following case.
You have an underwater engineering project where you have to pour, in a single pour, a concrete structure. If you pour too little, you will have to destroy the object and start over. If you end up with too much concrete, then you just discard the excess and never pour it. Just to make this interesting, let us make this an expensive event. Each cubic foot costs $1,000, and the true parameter is 1,000 cubic feet. If you purchase and pour 999 cubic feet, you have to destroy it, buy new concrete and start over. The cost is $999,000 in lost concrete plus demolition. If you purchase 1,001 cubic feet, your waste is $1,000.
If you choose quadratic loss to estimate the population parameter, half the time you will take catastrophic losses, if you use the true cost function created by errors, you can construct a weighted posterior point estimator that minimizes the average risk of loss.
There is nothing magic about the posterior mean. It should be used when your real loss from being wrong is quadratic. A quadratic loss is quite common in the real world, particularly in gambling. Remember this is not the loss created by gambling wrong, but by estimating the parameter wrong.
Is your real-world loss quadratic, if yes, then use the posterior mean, but if no then use the real loss function?
Bayesian solutions proper create an entire posterior density. If you are going to choose a point from it make sure your point makes sense to you. As a rule of thumb, you cannot average them as many do not have good compositional properties.
Now to the prior. Tim mentioned using hyperparameters as your alternative. That is taking the two parameters for the beta prior and creating functions to cover prior parameter uncertainty. It is a sort of prior averaging. Before you go there, you should think about what information you have. If you do not, then the good intrinsic properties of Bayesian methods go away, and the bias does become a problem.
Let us start with the Beta (0,0) prior. It has infinite mass at 0 and 1 and minimal mass at 50%. Do you really believe it is almost certainly exactly zero or one? Do you really believe it is unlikely to be one half? Make a picture of it; does it match your beliefs? The Beta (.5,.5), Jeffreys prior, does the same thing. The derivative is different, but there is still infinite weight on zero and one. Because they are symmetric priors, they also bias the expectation toward one half. Do you believe the true value of the parameter to have a fifty percent chance of being on either side of the one-half mark?
The Haldane prior was created for a real-world problem, the Beta (0,0). It is often the case in chemistry that you have to destroy your sample and you may always have a sample size of one. Is it soluble in water or is it not soluble in water (0-1)? Did it dissolve? Now you can make a proper statistical statement about it as you do have a prior. It is also the prior that maps to the Frequentist solution for the binomial. It is uninformative, but as you can see, you have three "uninformative priors." All priors convey information, even uninformative ones.
The Jeffreys prior was created to be invariant to monotonic transformations. That matters because probability statements can move with transformation. Only the maximum likelihood estimator is automatically invariant under transformation. It has more information in it than the Haldane prior and does not allow double-sided coins, which the Haldane prior does. Its virtue is that you can make transformations of the data without a consequence to your inference.
Finally, there is the uniform distribution, the Beta (1,1). Except for the case of a double-headed coin, it weights all possibilities equally.
Now here is the rub, do you really have no out-of-sample information about the true value of the parameter? For example, if you were doing bankruptcy research, you could estimate bankruptcy rates from loan interest rates. If bankruptcy rates are high, then loan rates would have to be higher to cover the risk and make a profit. Minimally, success is more likely than failure for seasoned businesses at least. So, knowing nothing else, you should have a Beta(1,2), the triangular distribution, $pi(theta)=1-theta$.
Now let us imagine you speak to a friend that is a bank loan officer and ask about loan losses and you are told it is 2%. Of course, that includes losses other than bankruptcy. Now you have a Beta(2,98), so $pi(theta)=9702theta(1-theta)^{97}$.
That is a lot of information, and you biased your outcome. That is a good thing because the bias isn't pure bias. It is information plus noise. It is the noise that you do not want, but cannot avoid. Look at the graph for this prior.
It is giving material weight to anything less than eight percent. It has reduced your search space and it has regularized the long run rate so that if you hit a run, such as bankruptcies in a major recession, it is normalized so that it will be perceived by the math as unlikely (forgive the anthropomorphization) and be underweighted.
More importantly, you have used professional information from outside your sample. That is perfectly good information. It is biased in that it is one bank's experience and you could certainly construct a better prior if you got more information and took more time to look.
Just as important, you preserved two properties that the Bayesian bias pays for, admissibility and coherence.
If you use your true prior then Bayesian methods cannot be stochastically dominated. Further, if you use your true prior then you can place fair bets and function as a bookie. You may not think of that as valuable, but a grocer is a bookie for fruit. They are gambling on inventory and placing a bet on how much you will buy and at what price. Frequentist methods are not coherent.
Now lets talk about the bad side of this prior, the one that worries you. Let us assume you collect a sample of 10,000 firms and over the period of time 10 go bankrupt. The sample bankruptcy rate is .1% but the posterior mean is .11%. Ha, you say, there is your bias. That is not the bias. The bias is from the true rate. If the true rate is .12% then it is biased downward. If it is .09% then it is biased upward.
You do not want to worry about the bias or getting the wrong prior. What you want to do is find the prior that works best for your problem, that is to say, it encodes your knowledge, whatever it may be, as closely as you can make a formula do that.
If you want an unbiased estimator do not use Bayesian methods. If you want to get an accurate estimator, encode your knowledge into the prior. If you have several possible priors, multiply them together and normalize them to one or use hyperparameters.
Part of your prior will be inaccurate just as a sample contains outliers or non-standardized components. Samples are bad too, you usually just cannot see it because you do not look. The prior regularizes and normalizes your sample.
No, please don't do that. The strength of Bayesian solutions is that they are subjective. The prior is a good thing and not something to be avoided. What you are doing is assuming the sample you are working with is a good sample, and your personal knowledge is of no value. It appears your fear is that of introducing bias.
All Bayesian methods are biased. That is not a bad thing. It is just a fact. As the sample size becomes very large, the Bayesian models become just barely biased if that helps.
So let us break apart some assumptions in your posting that may or may not have the results you intended. I am going to work back to front. You want to know about averaging posterior means.
The posterior mean minimizes quadratic loss. Unlike a Frequentist solution where this is the answer, this is not automatically true for Bayesian thought. To give you an example of where a posterior or a Frequentist mean would be a catastrophically bad idea consider the following case.
You have an underwater engineering project where you have to pour, in a single pour, a concrete structure. If you pour too little, you will have to destroy the object and start over. If you end up with too much concrete, then you just discard the excess and never pour it. Just to make this interesting, let us make this an expensive event. Each cubic foot costs $1,000, and the true parameter is 1,000 cubic feet. If you purchase and pour 999 cubic feet, you have to destroy it, buy new concrete and start over. The cost is $999,000 in lost concrete plus demolition. If you purchase 1,001 cubic feet, your waste is $1,000.
If you choose quadratic loss to estimate the population parameter, half the time you will take catastrophic losses, if you use the true cost function created by errors, you can construct a weighted posterior point estimator that minimizes the average risk of loss.
There is nothing magic about the posterior mean. It should be used when your real loss from being wrong is quadratic. A quadratic loss is quite common in the real world, particularly in gambling. Remember this is not the loss created by gambling wrong, but by estimating the parameter wrong.
Is your real-world loss quadratic, if yes, then use the posterior mean, but if no then use the real loss function?
Bayesian solutions proper create an entire posterior density. If you are going to choose a point from it make sure your point makes sense to you. As a rule of thumb, you cannot average them as many do not have good compositional properties.
Now to the prior. Tim mentioned using hyperparameters as your alternative. That is taking the two parameters for the beta prior and creating functions to cover prior parameter uncertainty. It is a sort of prior averaging. Before you go there, you should think about what information you have. If you do not, then the good intrinsic properties of Bayesian methods go away, and the bias does become a problem.
Let us start with the Beta (0,0) prior. It has infinite mass at 0 and 1 and minimal mass at 50%. Do you really believe it is almost certainly exactly zero or one? Do you really believe it is unlikely to be one half? Make a picture of it; does it match your beliefs? The Beta (.5,.5), Jeffreys prior, does the same thing. The derivative is different, but there is still infinite weight on zero and one. Because they are symmetric priors, they also bias the expectation toward one half. Do you believe the true value of the parameter to have a fifty percent chance of being on either side of the one-half mark?
The Haldane prior was created for a real-world problem, the Beta (0,0). It is often the case in chemistry that you have to destroy your sample and you may always have a sample size of one. Is it soluble in water or is it not soluble in water (0-1)? Did it dissolve? Now you can make a proper statistical statement about it as you do have a prior. It is also the prior that maps to the Frequentist solution for the binomial. It is uninformative, but as you can see, you have three "uninformative priors." All priors convey information, even uninformative ones.
The Jeffreys prior was created to be invariant to monotonic transformations. That matters because probability statements can move with transformation. Only the maximum likelihood estimator is automatically invariant under transformation. It has more information in it than the Haldane prior and does not allow double-sided coins, which the Haldane prior does. Its virtue is that you can make transformations of the data without a consequence to your inference.
Finally, there is the uniform distribution, the Beta (1,1). Except for the case of a double-headed coin, it weights all possibilities equally.
Now here is the rub, do you really have no out-of-sample information about the true value of the parameter? For example, if you were doing bankruptcy research, you could estimate bankruptcy rates from loan interest rates. If bankruptcy rates are high, then loan rates would have to be higher to cover the risk and make a profit. Minimally, success is more likely than failure for seasoned businesses at least. So, knowing nothing else, you should have a Beta(1,2), the triangular distribution, $pi(theta)=1-theta$.
Now let us imagine you speak to a friend that is a bank loan officer and ask about loan losses and you are told it is 2%. Of course, that includes losses other than bankruptcy. Now you have a Beta(2,98), so $pi(theta)=9702theta(1-theta)^{97}$.
That is a lot of information, and you biased your outcome. That is a good thing because the bias isn't pure bias. It is information plus noise. It is the noise that you do not want, but cannot avoid. Look at the graph for this prior.
It is giving material weight to anything less than eight percent. It has reduced your search space and it has regularized the long run rate so that if you hit a run, such as bankruptcies in a major recession, it is normalized so that it will be perceived by the math as unlikely (forgive the anthropomorphization) and be underweighted.
More importantly, you have used professional information from outside your sample. That is perfectly good information. It is biased in that it is one bank's experience and you could certainly construct a better prior if you got more information and took more time to look.
Just as important, you preserved two properties that the Bayesian bias pays for, admissibility and coherence.
If you use your true prior then Bayesian methods cannot be stochastically dominated. Further, if you use your true prior then you can place fair bets and function as a bookie. You may not think of that as valuable, but a grocer is a bookie for fruit. They are gambling on inventory and placing a bet on how much you will buy and at what price. Frequentist methods are not coherent.
Now lets talk about the bad side of this prior, the one that worries you. Let us assume you collect a sample of 10,000 firms and over the period of time 10 go bankrupt. The sample bankruptcy rate is .1% but the posterior mean is .11%. Ha, you say, there is your bias. That is not the bias. The bias is from the true rate. If the true rate is .12% then it is biased downward. If it is .09% then it is biased upward.
You do not want to worry about the bias or getting the wrong prior. What you want to do is find the prior that works best for your problem, that is to say, it encodes your knowledge, whatever it may be, as closely as you can make a formula do that.
If you want an unbiased estimator do not use Bayesian methods. If you want to get an accurate estimator, encode your knowledge into the prior. If you have several possible priors, multiply them together and normalize them to one or use hyperparameters.
Part of your prior will be inaccurate just as a sample contains outliers or non-standardized components. Samples are bad too, you usually just cannot see it because you do not look. The prior regularizes and normalizes your sample.
answered Dec 6 at 17:22
Dave Harris
3,359415
3,359415
1
If I could give you 100 upvotes, I would. Thank you for the detailed answer. You answered all my questions. Thank you Kindly!!!
– Humean David
Dec 6 at 17:26
@HumeanDavid, then you should also accept his answer :)
– Avraham
Dec 6 at 22:55
add a comment |
1
If I could give you 100 upvotes, I would. Thank you for the detailed answer. You answered all my questions. Thank you Kindly!!!
– Humean David
Dec 6 at 17:26
@HumeanDavid, then you should also accept his answer :)
– Avraham
Dec 6 at 22:55
1
1
If I could give you 100 upvotes, I would. Thank you for the detailed answer. You answered all my questions. Thank you Kindly!!!
– Humean David
Dec 6 at 17:26
If I could give you 100 upvotes, I would. Thank you for the detailed answer. You answered all my questions. Thank you Kindly!!!
– Humean David
Dec 6 at 17:26
@HumeanDavid, then you should also accept his answer :)
– Avraham
Dec 6 at 22:55
@HumeanDavid, then you should also accept his answer :)
– Avraham
Dec 6 at 22:55
add a comment |
up vote
7
down vote
The fundamental difficulty with averaging the posteriors is that the data has no say in the weight of the said posteriors. If the global prior is the average of three possible priors (with weights $1/3$, $1/3$, $1/3$), the posterior will not be the average of the corresponding posteriors with weights $1/3$, $1/3$, $1/3$, as the weights will depend on the data.
Check out the keyword Bayesian model averaging
for more details. As for instance in Hoeting et al. (1999). Or these slides.
1
It’s worth mentioning that, just because naive averaging disagrees with BMA, this does not mean naive averaging will actually perform worse in practice. For example, my experience is that Bayesian CART often performs worse than naively sampling decision trees from the prior and averaging together the associated predictions. This is in terms of prediction error on heldout data, say.
– guy
Dec 6 at 22:56
add a comment |
up vote
7
down vote
The fundamental difficulty with averaging the posteriors is that the data has no say in the weight of the said posteriors. If the global prior is the average of three possible priors (with weights $1/3$, $1/3$, $1/3$), the posterior will not be the average of the corresponding posteriors with weights $1/3$, $1/3$, $1/3$, as the weights will depend on the data.
Check out the keyword Bayesian model averaging
for more details. As for instance in Hoeting et al. (1999). Or these slides.
1
It’s worth mentioning that, just because naive averaging disagrees with BMA, this does not mean naive averaging will actually perform worse in practice. For example, my experience is that Bayesian CART often performs worse than naively sampling decision trees from the prior and averaging together the associated predictions. This is in terms of prediction error on heldout data, say.
– guy
Dec 6 at 22:56
add a comment |
up vote
7
down vote
up vote
7
down vote
The fundamental difficulty with averaging the posteriors is that the data has no say in the weight of the said posteriors. If the global prior is the average of three possible priors (with weights $1/3$, $1/3$, $1/3$), the posterior will not be the average of the corresponding posteriors with weights $1/3$, $1/3$, $1/3$, as the weights will depend on the data.
Check out the keyword Bayesian model averaging
for more details. As for instance in Hoeting et al. (1999). Or these slides.
The fundamental difficulty with averaging the posteriors is that the data has no say in the weight of the said posteriors. If the global prior is the average of three possible priors (with weights $1/3$, $1/3$, $1/3$), the posterior will not be the average of the corresponding posteriors with weights $1/3$, $1/3$, $1/3$, as the weights will depend on the data.
Check out the keyword Bayesian model averaging
for more details. As for instance in Hoeting et al. (1999). Or these slides.
edited Dec 6 at 21:47
answered Dec 6 at 16:11


Xi'an
52.8k688340
52.8k688340
1
It’s worth mentioning that, just because naive averaging disagrees with BMA, this does not mean naive averaging will actually perform worse in practice. For example, my experience is that Bayesian CART often performs worse than naively sampling decision trees from the prior and averaging together the associated predictions. This is in terms of prediction error on heldout data, say.
– guy
Dec 6 at 22:56
add a comment |
1
It’s worth mentioning that, just because naive averaging disagrees with BMA, this does not mean naive averaging will actually perform worse in practice. For example, my experience is that Bayesian CART often performs worse than naively sampling decision trees from the prior and averaging together the associated predictions. This is in terms of prediction error on heldout data, say.
– guy
Dec 6 at 22:56
1
1
It’s worth mentioning that, just because naive averaging disagrees with BMA, this does not mean naive averaging will actually perform worse in practice. For example, my experience is that Bayesian CART often performs worse than naively sampling decision trees from the prior and averaging together the associated predictions. This is in terms of prediction error on heldout data, say.
– guy
Dec 6 at 22:56
It’s worth mentioning that, just because naive averaging disagrees with BMA, this does not mean naive averaging will actually perform worse in practice. For example, my experience is that Bayesian CART often performs worse than naively sampling decision trees from the prior and averaging together the associated predictions. This is in terms of prediction error on heldout data, say.
– guy
Dec 6 at 22:56
add a comment |
up vote
1
down vote
Assume your posterior is a multimodal distribution. If your different priors are chosen such that you only get one region of high probability per prior, then your average of the means would lie somewhere between these means, but in probably in a region with low mass.
So better not taking the average of means.
New contributor
Balou is a new contributor to this site. Take care in asking for clarification, commenting, and answering.
Check out our Code of Conduct.
add a comment |
up vote
1
down vote
Assume your posterior is a multimodal distribution. If your different priors are chosen such that you only get one region of high probability per prior, then your average of the means would lie somewhere between these means, but in probably in a region with low mass.
So better not taking the average of means.
New contributor
Balou is a new contributor to this site. Take care in asking for clarification, commenting, and answering.
Check out our Code of Conduct.
add a comment |
up vote
1
down vote
up vote
1
down vote
Assume your posterior is a multimodal distribution. If your different priors are chosen such that you only get one region of high probability per prior, then your average of the means would lie somewhere between these means, but in probably in a region with low mass.
So better not taking the average of means.
New contributor
Balou is a new contributor to this site. Take care in asking for clarification, commenting, and answering.
Check out our Code of Conduct.
Assume your posterior is a multimodal distribution. If your different priors are chosen such that you only get one region of high probability per prior, then your average of the means would lie somewhere between these means, but in probably in a region with low mass.
So better not taking the average of means.
New contributor
Balou is a new contributor to this site. Take care in asking for clarification, commenting, and answering.
Check out our Code of Conduct.
New contributor
Balou is a new contributor to this site. Take care in asking for clarification, commenting, and answering.
Check out our Code of Conduct.
answered Dec 6 at 16:56
Balou
213
213
New contributor
Balou is a new contributor to this site. Take care in asking for clarification, commenting, and answering.
Check out our Code of Conduct.
New contributor
Balou is a new contributor to this site. Take care in asking for clarification, commenting, and answering.
Check out our Code of Conduct.
Balou is a new contributor to this site. Take care in asking for clarification, commenting, and answering.
Check out our Code of Conduct.
add a comment |
add a comment |
h3lR1,U fS,gXJfq0
4
If you cannot pick the priors, why not using hyperpriors for the parameter of the priors? This is what is usually done in Bayesian setting.
– Tim♦
Dec 6 at 12:50
Hi Tim, thank you. Can you please expand? What would be the hyperprior for, let’s say uniform prior (1, 1)?
– Humean David
Dec 6 at 13:31
1
Averaging what? If you average returned probabilities, they may not sum/integrate to one any more. Why averaging three arbitrary priors would solve anything? Why not two, or 62 different priors? Using hyperpriors does exactly that: it lets you integrate over a whole range of possible priors.
– Tim♦
Dec 6 at 14:51
1
Since, as I said, using hyperpriors seem to do exactly the same thing but using method that (a) is pretty standard in Bayesian setting, (b) has theoretical justification within the Bayesian framework, so why instead would you like to use arbitrary method, that potentially gives you uninterpretable results (probabilities that do not sum to one)?
– Tim♦
Dec 6 at 15:49
1
Is OP referring to model averaging? Model averaging is not motivated by uncertainty in parameters for priors. Like @Tim said, use a hyperprior. It is motivated by the uncertainty inherent to any modeling exercise. You can use something like the WAIC to create weights for each candidate model, then compute weighted averages.
– Heteroskedastic Jim
Dec 6 at 17:21