Is stochastic gradient descent pseudo-stochastic?
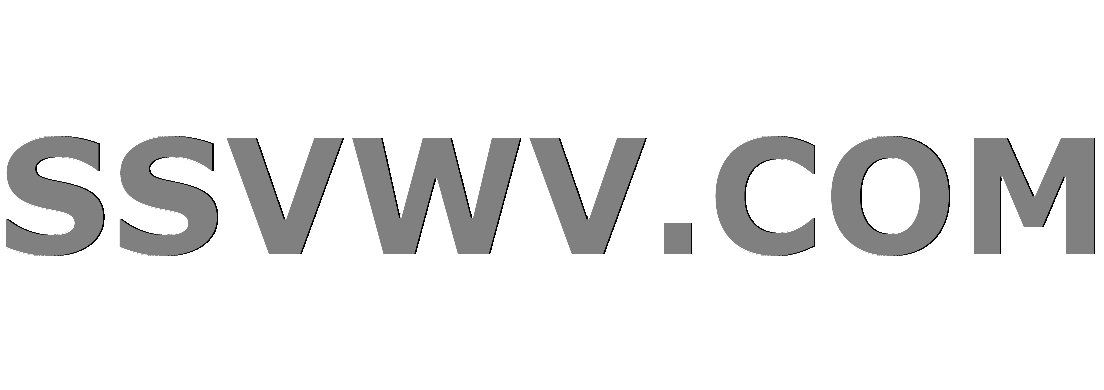
Multi tool use
$begingroup$
I know that stochastic gradient descent randomly chooses 1 sample to update the weights. An epoch is defined as using all $N$ samples. So with SGD, for each epoch, we update the weights $N$ times.
My confusion is doesn't this make it so you have to go through all $N$ samples before you can see the same sample twice? Doesn't that effectively make it pseudo-random/stochastic? If it was entirely random, then there would be a possibility of seeing the same sample more than once before going through all $N$ samples.
machine-learning neural-networks gradient-descent sgd
$endgroup$
add a comment |
$begingroup$
I know that stochastic gradient descent randomly chooses 1 sample to update the weights. An epoch is defined as using all $N$ samples. So with SGD, for each epoch, we update the weights $N$ times.
My confusion is doesn't this make it so you have to go through all $N$ samples before you can see the same sample twice? Doesn't that effectively make it pseudo-random/stochastic? If it was entirely random, then there would be a possibility of seeing the same sample more than once before going through all $N$ samples.
machine-learning neural-networks gradient-descent sgd
$endgroup$
add a comment |
$begingroup$
I know that stochastic gradient descent randomly chooses 1 sample to update the weights. An epoch is defined as using all $N$ samples. So with SGD, for each epoch, we update the weights $N$ times.
My confusion is doesn't this make it so you have to go through all $N$ samples before you can see the same sample twice? Doesn't that effectively make it pseudo-random/stochastic? If it was entirely random, then there would be a possibility of seeing the same sample more than once before going through all $N$ samples.
machine-learning neural-networks gradient-descent sgd
$endgroup$
I know that stochastic gradient descent randomly chooses 1 sample to update the weights. An epoch is defined as using all $N$ samples. So with SGD, for each epoch, we update the weights $N$ times.
My confusion is doesn't this make it so you have to go through all $N$ samples before you can see the same sample twice? Doesn't that effectively make it pseudo-random/stochastic? If it was entirely random, then there would be a possibility of seeing the same sample more than once before going through all $N$ samples.
machine-learning neural-networks gradient-descent sgd
machine-learning neural-networks gradient-descent sgd
edited yesterday


Sycorax
41.9k12109206
41.9k12109206
asked yesterday
IamanonIamanon
303
303
add a comment |
add a comment |
1 Answer
1
active
oldest
votes
$begingroup$
Exhausting all $N$ samples before being able to repeat a sample means that the process is not independent. However, the process is still stochastic.
Consider a shuffled deck of cards. You look at the top card and see $mathsf{A}spadesuit$ (Ace of Spades), and set it aside. You'll never see another $mathsf{A}spadesuit$ in the whole deck. However, you don't know anything about the ordering of the remaining 51 cards, because the deck is shuffled. In this sense, the remainder of the deck still has a random order. The next card could be a $mathsf{2}color{red}{heartsuit}$ or $mathsf{J}clubsuit$. You don't know for sure; all you do know is that the next card isn't the Ace of Spades, because you've put the only $mathsf{A}spadesuit$ face-up somewhere else.
In the scenario you outline, you're suggesting looking at the top card and then shuffling it into the deck again. This implies that the probability of seeing the $mathsf{A}spadesuit$ is independent of the previously-observed cards. Independence of events is an important attribute in probability theory, but it is not required to define a random process.
You might wonder why a person would want to construct mini-batches using the non-independent strategy. That question is answered here: Why do neural network researchers care about epochs?
$endgroup$
add a comment |
Your Answer
StackExchange.ifUsing("editor", function () {
return StackExchange.using("mathjaxEditing", function () {
StackExchange.MarkdownEditor.creationCallbacks.add(function (editor, postfix) {
StackExchange.mathjaxEditing.prepareWmdForMathJax(editor, postfix, [["$", "$"], ["\\(","\\)"]]);
});
});
}, "mathjax-editing");
StackExchange.ready(function() {
var channelOptions = {
tags: "".split(" "),
id: "65"
};
initTagRenderer("".split(" "), "".split(" "), channelOptions);
StackExchange.using("externalEditor", function() {
// Have to fire editor after snippets, if snippets enabled
if (StackExchange.settings.snippets.snippetsEnabled) {
StackExchange.using("snippets", function() {
createEditor();
});
}
else {
createEditor();
}
});
function createEditor() {
StackExchange.prepareEditor({
heartbeatType: 'answer',
autoActivateHeartbeat: false,
convertImagesToLinks: false,
noModals: true,
showLowRepImageUploadWarning: true,
reputationToPostImages: null,
bindNavPrevention: true,
postfix: "",
imageUploader: {
brandingHtml: "Powered by u003ca class="icon-imgur-white" href="https://imgur.com/"u003eu003c/au003e",
contentPolicyHtml: "User contributions licensed under u003ca href="https://creativecommons.org/licenses/by-sa/3.0/"u003ecc by-sa 3.0 with attribution requiredu003c/au003e u003ca href="https://stackoverflow.com/legal/content-policy"u003e(content policy)u003c/au003e",
allowUrls: true
},
onDemand: true,
discardSelector: ".discard-answer"
,immediatelyShowMarkdownHelp:true
});
}
});
Sign up or log in
StackExchange.ready(function () {
StackExchange.helpers.onClickDraftSave('#login-link');
});
Sign up using Google
Sign up using Facebook
Sign up using Email and Password
Post as a guest
Required, but never shown
StackExchange.ready(
function () {
StackExchange.openid.initPostLogin('.new-post-login', 'https%3a%2f%2fstats.stackexchange.com%2fquestions%2f398540%2fis-stochastic-gradient-descent-pseudo-stochastic%23new-answer', 'question_page');
}
);
Post as a guest
Required, but never shown
1 Answer
1
active
oldest
votes
1 Answer
1
active
oldest
votes
active
oldest
votes
active
oldest
votes
$begingroup$
Exhausting all $N$ samples before being able to repeat a sample means that the process is not independent. However, the process is still stochastic.
Consider a shuffled deck of cards. You look at the top card and see $mathsf{A}spadesuit$ (Ace of Spades), and set it aside. You'll never see another $mathsf{A}spadesuit$ in the whole deck. However, you don't know anything about the ordering of the remaining 51 cards, because the deck is shuffled. In this sense, the remainder of the deck still has a random order. The next card could be a $mathsf{2}color{red}{heartsuit}$ or $mathsf{J}clubsuit$. You don't know for sure; all you do know is that the next card isn't the Ace of Spades, because you've put the only $mathsf{A}spadesuit$ face-up somewhere else.
In the scenario you outline, you're suggesting looking at the top card and then shuffling it into the deck again. This implies that the probability of seeing the $mathsf{A}spadesuit$ is independent of the previously-observed cards. Independence of events is an important attribute in probability theory, but it is not required to define a random process.
You might wonder why a person would want to construct mini-batches using the non-independent strategy. That question is answered here: Why do neural network researchers care about epochs?
$endgroup$
add a comment |
$begingroup$
Exhausting all $N$ samples before being able to repeat a sample means that the process is not independent. However, the process is still stochastic.
Consider a shuffled deck of cards. You look at the top card and see $mathsf{A}spadesuit$ (Ace of Spades), and set it aside. You'll never see another $mathsf{A}spadesuit$ in the whole deck. However, you don't know anything about the ordering of the remaining 51 cards, because the deck is shuffled. In this sense, the remainder of the deck still has a random order. The next card could be a $mathsf{2}color{red}{heartsuit}$ or $mathsf{J}clubsuit$. You don't know for sure; all you do know is that the next card isn't the Ace of Spades, because you've put the only $mathsf{A}spadesuit$ face-up somewhere else.
In the scenario you outline, you're suggesting looking at the top card and then shuffling it into the deck again. This implies that the probability of seeing the $mathsf{A}spadesuit$ is independent of the previously-observed cards. Independence of events is an important attribute in probability theory, but it is not required to define a random process.
You might wonder why a person would want to construct mini-batches using the non-independent strategy. That question is answered here: Why do neural network researchers care about epochs?
$endgroup$
add a comment |
$begingroup$
Exhausting all $N$ samples before being able to repeat a sample means that the process is not independent. However, the process is still stochastic.
Consider a shuffled deck of cards. You look at the top card and see $mathsf{A}spadesuit$ (Ace of Spades), and set it aside. You'll never see another $mathsf{A}spadesuit$ in the whole deck. However, you don't know anything about the ordering of the remaining 51 cards, because the deck is shuffled. In this sense, the remainder of the deck still has a random order. The next card could be a $mathsf{2}color{red}{heartsuit}$ or $mathsf{J}clubsuit$. You don't know for sure; all you do know is that the next card isn't the Ace of Spades, because you've put the only $mathsf{A}spadesuit$ face-up somewhere else.
In the scenario you outline, you're suggesting looking at the top card and then shuffling it into the deck again. This implies that the probability of seeing the $mathsf{A}spadesuit$ is independent of the previously-observed cards. Independence of events is an important attribute in probability theory, but it is not required to define a random process.
You might wonder why a person would want to construct mini-batches using the non-independent strategy. That question is answered here: Why do neural network researchers care about epochs?
$endgroup$
Exhausting all $N$ samples before being able to repeat a sample means that the process is not independent. However, the process is still stochastic.
Consider a shuffled deck of cards. You look at the top card and see $mathsf{A}spadesuit$ (Ace of Spades), and set it aside. You'll never see another $mathsf{A}spadesuit$ in the whole deck. However, you don't know anything about the ordering of the remaining 51 cards, because the deck is shuffled. In this sense, the remainder of the deck still has a random order. The next card could be a $mathsf{2}color{red}{heartsuit}$ or $mathsf{J}clubsuit$. You don't know for sure; all you do know is that the next card isn't the Ace of Spades, because you've put the only $mathsf{A}spadesuit$ face-up somewhere else.
In the scenario you outline, you're suggesting looking at the top card and then shuffling it into the deck again. This implies that the probability of seeing the $mathsf{A}spadesuit$ is independent of the previously-observed cards. Independence of events is an important attribute in probability theory, but it is not required to define a random process.
You might wonder why a person would want to construct mini-batches using the non-independent strategy. That question is answered here: Why do neural network researchers care about epochs?
edited yesterday
answered yesterday


SycoraxSycorax
41.9k12109206
41.9k12109206
add a comment |
add a comment |
Thanks for contributing an answer to Cross Validated!
- Please be sure to answer the question. Provide details and share your research!
But avoid …
- Asking for help, clarification, or responding to other answers.
- Making statements based on opinion; back them up with references or personal experience.
Use MathJax to format equations. MathJax reference.
To learn more, see our tips on writing great answers.
Sign up or log in
StackExchange.ready(function () {
StackExchange.helpers.onClickDraftSave('#login-link');
});
Sign up using Google
Sign up using Facebook
Sign up using Email and Password
Post as a guest
Required, but never shown
StackExchange.ready(
function () {
StackExchange.openid.initPostLogin('.new-post-login', 'https%3a%2f%2fstats.stackexchange.com%2fquestions%2f398540%2fis-stochastic-gradient-descent-pseudo-stochastic%23new-answer', 'question_page');
}
);
Post as a guest
Required, but never shown
Sign up or log in
StackExchange.ready(function () {
StackExchange.helpers.onClickDraftSave('#login-link');
});
Sign up using Google
Sign up using Facebook
Sign up using Email and Password
Post as a guest
Required, but never shown
Sign up or log in
StackExchange.ready(function () {
StackExchange.helpers.onClickDraftSave('#login-link');
});
Sign up using Google
Sign up using Facebook
Sign up using Email and Password
Post as a guest
Required, but never shown
Sign up or log in
StackExchange.ready(function () {
StackExchange.helpers.onClickDraftSave('#login-link');
});
Sign up using Google
Sign up using Facebook
Sign up using Email and Password
Sign up using Google
Sign up using Facebook
Sign up using Email and Password
Post as a guest
Required, but never shown
Required, but never shown
Required, but never shown
Required, but never shown
Required, but never shown
Required, but never shown
Required, but never shown
Required, but never shown
Required, but never shown
jx9PyjWmrac 1