Determining multivariate least squares with constraint
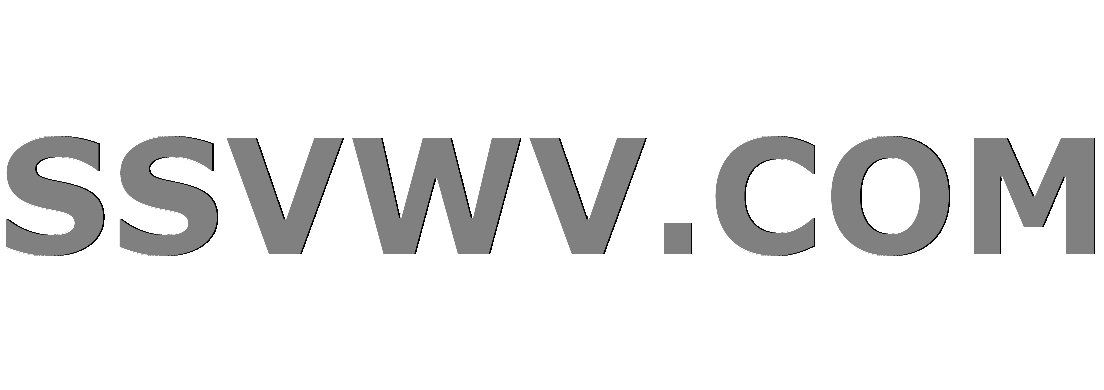
Multi tool use
$begingroup$
I have some composition data for a geological sample and its constituent minerals. I want to estimate the proportions of the minerals that make up the bulk sample. This is essentially a mass balance problem. Each variable is pseudo-independent (but constrained by constant sum).
I'm starting with the matrix form $a.x=b$,
where, $a$ is the array $elemental~composition~vector times mineral ~species$, $x$ is the column vector of mineral proportions, and $b$ is the bulk composition. The key constraint with this kind of problem is that
$0 leq x_{j} leq 1~~~~~(j=1,...m)$
and
$sum_{j=1}^m x_{j}=1$
here is some example data
a={{63.1545, 64.3049, 100., 37.1417, 32.4026, 30.0382, 30.7033, 0., 36.5444, 0., 0., 0., 0.0034},
{0.0016, 0.01, 0., 0.6641, 2.35946, 26.91, 0., 0., 0.297125, 0., 0., 0., 51.3026},
{17.8683, 21.096, 0., 11.2387, 14.3019, 6.46115, 0.34805, 0., 14.368, 0., 0., 0., 0.},
{0.0223, 0.1191, 0., 30.6859, 32.0779, 1.5471, 1.5014, 0., 11.5299, 0., 0., 0., 43.3621},
{0., 0., 0., 0.94408, 0.6131, 0.0986, 0., 0., 0., 0., 0., 0., 2.1039},
{0., 0., 0., 1.14598, 1.82486, 0.03695, 0.00935, 0., 0.2312, 0., 0., 0., 0.0166},
{0.625667, 9.77073, 0., 1.35584, 0.0558, 0.05635, 0., 0., 0.079275, 0., 0., 0., 0.018},
{0., 2.80603, 0., 10.7388, 0.0245429, 27.7454, 2.19443, 55.08, 10.5478, 56.03, 78.13, 10.44, 0.0262},
{15.7365, 0.0874667, 0., 1.89652, 8.54851, 0.00475, 0., 0., 0.09665, 0., 0., 0., 0.0115},
{0., 0., 0., 0.16636, 0., 0., 0.0293, 42.4, 0.0114, 0., 0., 0., 0.0617}}
b={65.67, 0.52, 14.77, 5.418, 0.13, 0.3, 3.22, 2.05, 6.07, 0.13}
If I use the LeastSquares
function I get
lsq = LeastSquares[a, b]
{0.331681, 0.283916, 0.166439, 0.172393, 0.0586919,
0.0795275,
0.00802007, 0.00244529, -0.030593, -0.0157832, -0.0220087,
-0.00294087, -0.0363984}
This result looks OK, except negative proportions are not allowed and the sum does not equal 1.
Total@lsq
0.99539
How do I implement linear least squares fitting for multivariate data under the constraint that values lie between 0 to 1 and sum to 1 in Mathematica?
Also how can I derive the residuals for the fit?
fitting
$endgroup$
|
show 2 more comments
$begingroup$
I have some composition data for a geological sample and its constituent minerals. I want to estimate the proportions of the minerals that make up the bulk sample. This is essentially a mass balance problem. Each variable is pseudo-independent (but constrained by constant sum).
I'm starting with the matrix form $a.x=b$,
where, $a$ is the array $elemental~composition~vector times mineral ~species$, $x$ is the column vector of mineral proportions, and $b$ is the bulk composition. The key constraint with this kind of problem is that
$0 leq x_{j} leq 1~~~~~(j=1,...m)$
and
$sum_{j=1}^m x_{j}=1$
here is some example data
a={{63.1545, 64.3049, 100., 37.1417, 32.4026, 30.0382, 30.7033, 0., 36.5444, 0., 0., 0., 0.0034},
{0.0016, 0.01, 0., 0.6641, 2.35946, 26.91, 0., 0., 0.297125, 0., 0., 0., 51.3026},
{17.8683, 21.096, 0., 11.2387, 14.3019, 6.46115, 0.34805, 0., 14.368, 0., 0., 0., 0.},
{0.0223, 0.1191, 0., 30.6859, 32.0779, 1.5471, 1.5014, 0., 11.5299, 0., 0., 0., 43.3621},
{0., 0., 0., 0.94408, 0.6131, 0.0986, 0., 0., 0., 0., 0., 0., 2.1039},
{0., 0., 0., 1.14598, 1.82486, 0.03695, 0.00935, 0., 0.2312, 0., 0., 0., 0.0166},
{0.625667, 9.77073, 0., 1.35584, 0.0558, 0.05635, 0., 0., 0.079275, 0., 0., 0., 0.018},
{0., 2.80603, 0., 10.7388, 0.0245429, 27.7454, 2.19443, 55.08, 10.5478, 56.03, 78.13, 10.44, 0.0262},
{15.7365, 0.0874667, 0., 1.89652, 8.54851, 0.00475, 0., 0., 0.09665, 0., 0., 0., 0.0115},
{0., 0., 0., 0.16636, 0., 0., 0.0293, 42.4, 0.0114, 0., 0., 0., 0.0617}}
b={65.67, 0.52, 14.77, 5.418, 0.13, 0.3, 3.22, 2.05, 6.07, 0.13}
If I use the LeastSquares
function I get
lsq = LeastSquares[a, b]
{0.331681, 0.283916, 0.166439, 0.172393, 0.0586919,
0.0795275,
0.00802007, 0.00244529, -0.030593, -0.0157832, -0.0220087,
-0.00294087, -0.0363984}
This result looks OK, except negative proportions are not allowed and the sum does not equal 1.
Total@lsq
0.99539
How do I implement linear least squares fitting for multivariate data under the constraint that values lie between 0 to 1 and sum to 1 in Mathematica?
Also how can I derive the residuals for the fit?
fitting
$endgroup$
2
$begingroup$
My usual soapbox speech: Why not consult a statistician first and then figure out how to implement an appropriate process in Mathematica? Consider stats.stackexchange.com/questions/267014/….
$endgroup$
– JimB
yesterday
$begingroup$
@JimB precisely because someone will tell me that I can't use continuum methods with compositional data. The constant sum problem is considered a vague oddity in geological circles and largely ignored (for better or worse).
$endgroup$
– geordie
yesterday
$begingroup$
I'm sure there must be more to it than "They won't let me do what I want!" Compositional data analysis has a pretty standard set of statistical approaches. Chemists use it all the time. These approaches are many times called "mixture designs": itl.nist.gov/div898/handbook/pri/section5/pri54.htm. Maybe you've been talking to the wrong folks.
$endgroup$
– JimB
yesterday
$begingroup$
Like I said, geologists don't. Perhaps it has to do with the general 'noisiness' of geoscience datasets. Most other sciences are able to rely on fairly precise numbers.
$endgroup$
– geordie
yesterday
1
$begingroup$
"Most other sciences are able to rely on fairly precise numbers." Ha, ha. You're funny!
$endgroup$
– JimB
yesterday
|
show 2 more comments
$begingroup$
I have some composition data for a geological sample and its constituent minerals. I want to estimate the proportions of the minerals that make up the bulk sample. This is essentially a mass balance problem. Each variable is pseudo-independent (but constrained by constant sum).
I'm starting with the matrix form $a.x=b$,
where, $a$ is the array $elemental~composition~vector times mineral ~species$, $x$ is the column vector of mineral proportions, and $b$ is the bulk composition. The key constraint with this kind of problem is that
$0 leq x_{j} leq 1~~~~~(j=1,...m)$
and
$sum_{j=1}^m x_{j}=1$
here is some example data
a={{63.1545, 64.3049, 100., 37.1417, 32.4026, 30.0382, 30.7033, 0., 36.5444, 0., 0., 0., 0.0034},
{0.0016, 0.01, 0., 0.6641, 2.35946, 26.91, 0., 0., 0.297125, 0., 0., 0., 51.3026},
{17.8683, 21.096, 0., 11.2387, 14.3019, 6.46115, 0.34805, 0., 14.368, 0., 0., 0., 0.},
{0.0223, 0.1191, 0., 30.6859, 32.0779, 1.5471, 1.5014, 0., 11.5299, 0., 0., 0., 43.3621},
{0., 0., 0., 0.94408, 0.6131, 0.0986, 0., 0., 0., 0., 0., 0., 2.1039},
{0., 0., 0., 1.14598, 1.82486, 0.03695, 0.00935, 0., 0.2312, 0., 0., 0., 0.0166},
{0.625667, 9.77073, 0., 1.35584, 0.0558, 0.05635, 0., 0., 0.079275, 0., 0., 0., 0.018},
{0., 2.80603, 0., 10.7388, 0.0245429, 27.7454, 2.19443, 55.08, 10.5478, 56.03, 78.13, 10.44, 0.0262},
{15.7365, 0.0874667, 0., 1.89652, 8.54851, 0.00475, 0., 0., 0.09665, 0., 0., 0., 0.0115},
{0., 0., 0., 0.16636, 0., 0., 0.0293, 42.4, 0.0114, 0., 0., 0., 0.0617}}
b={65.67, 0.52, 14.77, 5.418, 0.13, 0.3, 3.22, 2.05, 6.07, 0.13}
If I use the LeastSquares
function I get
lsq = LeastSquares[a, b]
{0.331681, 0.283916, 0.166439, 0.172393, 0.0586919,
0.0795275,
0.00802007, 0.00244529, -0.030593, -0.0157832, -0.0220087,
-0.00294087, -0.0363984}
This result looks OK, except negative proportions are not allowed and the sum does not equal 1.
Total@lsq
0.99539
How do I implement linear least squares fitting for multivariate data under the constraint that values lie between 0 to 1 and sum to 1 in Mathematica?
Also how can I derive the residuals for the fit?
fitting
$endgroup$
I have some composition data for a geological sample and its constituent minerals. I want to estimate the proportions of the minerals that make up the bulk sample. This is essentially a mass balance problem. Each variable is pseudo-independent (but constrained by constant sum).
I'm starting with the matrix form $a.x=b$,
where, $a$ is the array $elemental~composition~vector times mineral ~species$, $x$ is the column vector of mineral proportions, and $b$ is the bulk composition. The key constraint with this kind of problem is that
$0 leq x_{j} leq 1~~~~~(j=1,...m)$
and
$sum_{j=1}^m x_{j}=1$
here is some example data
a={{63.1545, 64.3049, 100., 37.1417, 32.4026, 30.0382, 30.7033, 0., 36.5444, 0., 0., 0., 0.0034},
{0.0016, 0.01, 0., 0.6641, 2.35946, 26.91, 0., 0., 0.297125, 0., 0., 0., 51.3026},
{17.8683, 21.096, 0., 11.2387, 14.3019, 6.46115, 0.34805, 0., 14.368, 0., 0., 0., 0.},
{0.0223, 0.1191, 0., 30.6859, 32.0779, 1.5471, 1.5014, 0., 11.5299, 0., 0., 0., 43.3621},
{0., 0., 0., 0.94408, 0.6131, 0.0986, 0., 0., 0., 0., 0., 0., 2.1039},
{0., 0., 0., 1.14598, 1.82486, 0.03695, 0.00935, 0., 0.2312, 0., 0., 0., 0.0166},
{0.625667, 9.77073, 0., 1.35584, 0.0558, 0.05635, 0., 0., 0.079275, 0., 0., 0., 0.018},
{0., 2.80603, 0., 10.7388, 0.0245429, 27.7454, 2.19443, 55.08, 10.5478, 56.03, 78.13, 10.44, 0.0262},
{15.7365, 0.0874667, 0., 1.89652, 8.54851, 0.00475, 0., 0., 0.09665, 0., 0., 0., 0.0115},
{0., 0., 0., 0.16636, 0., 0., 0.0293, 42.4, 0.0114, 0., 0., 0., 0.0617}}
b={65.67, 0.52, 14.77, 5.418, 0.13, 0.3, 3.22, 2.05, 6.07, 0.13}
If I use the LeastSquares
function I get
lsq = LeastSquares[a, b]
{0.331681, 0.283916, 0.166439, 0.172393, 0.0586919,
0.0795275,
0.00802007, 0.00244529, -0.030593, -0.0157832, -0.0220087,
-0.00294087, -0.0363984}
This result looks OK, except negative proportions are not allowed and the sum does not equal 1.
Total@lsq
0.99539
How do I implement linear least squares fitting for multivariate data under the constraint that values lie between 0 to 1 and sum to 1 in Mathematica?
Also how can I derive the residuals for the fit?
fitting
fitting
edited yesterday
user64494
3,55311022
3,55311022
asked yesterday


geordiegeordie
2,0491630
2,0491630
2
$begingroup$
My usual soapbox speech: Why not consult a statistician first and then figure out how to implement an appropriate process in Mathematica? Consider stats.stackexchange.com/questions/267014/….
$endgroup$
– JimB
yesterday
$begingroup$
@JimB precisely because someone will tell me that I can't use continuum methods with compositional data. The constant sum problem is considered a vague oddity in geological circles and largely ignored (for better or worse).
$endgroup$
– geordie
yesterday
$begingroup$
I'm sure there must be more to it than "They won't let me do what I want!" Compositional data analysis has a pretty standard set of statistical approaches. Chemists use it all the time. These approaches are many times called "mixture designs": itl.nist.gov/div898/handbook/pri/section5/pri54.htm. Maybe you've been talking to the wrong folks.
$endgroup$
– JimB
yesterday
$begingroup$
Like I said, geologists don't. Perhaps it has to do with the general 'noisiness' of geoscience datasets. Most other sciences are able to rely on fairly precise numbers.
$endgroup$
– geordie
yesterday
1
$begingroup$
"Most other sciences are able to rely on fairly precise numbers." Ha, ha. You're funny!
$endgroup$
– JimB
yesterday
|
show 2 more comments
2
$begingroup$
My usual soapbox speech: Why not consult a statistician first and then figure out how to implement an appropriate process in Mathematica? Consider stats.stackexchange.com/questions/267014/….
$endgroup$
– JimB
yesterday
$begingroup$
@JimB precisely because someone will tell me that I can't use continuum methods with compositional data. The constant sum problem is considered a vague oddity in geological circles and largely ignored (for better or worse).
$endgroup$
– geordie
yesterday
$begingroup$
I'm sure there must be more to it than "They won't let me do what I want!" Compositional data analysis has a pretty standard set of statistical approaches. Chemists use it all the time. These approaches are many times called "mixture designs": itl.nist.gov/div898/handbook/pri/section5/pri54.htm. Maybe you've been talking to the wrong folks.
$endgroup$
– JimB
yesterday
$begingroup$
Like I said, geologists don't. Perhaps it has to do with the general 'noisiness' of geoscience datasets. Most other sciences are able to rely on fairly precise numbers.
$endgroup$
– geordie
yesterday
1
$begingroup$
"Most other sciences are able to rely on fairly precise numbers." Ha, ha. You're funny!
$endgroup$
– JimB
yesterday
2
2
$begingroup$
My usual soapbox speech: Why not consult a statistician first and then figure out how to implement an appropriate process in Mathematica? Consider stats.stackexchange.com/questions/267014/….
$endgroup$
– JimB
yesterday
$begingroup$
My usual soapbox speech: Why not consult a statistician first and then figure out how to implement an appropriate process in Mathematica? Consider stats.stackexchange.com/questions/267014/….
$endgroup$
– JimB
yesterday
$begingroup$
@JimB precisely because someone will tell me that I can't use continuum methods with compositional data. The constant sum problem is considered a vague oddity in geological circles and largely ignored (for better or worse).
$endgroup$
– geordie
yesterday
$begingroup$
@JimB precisely because someone will tell me that I can't use continuum methods with compositional data. The constant sum problem is considered a vague oddity in geological circles and largely ignored (for better or worse).
$endgroup$
– geordie
yesterday
$begingroup$
I'm sure there must be more to it than "They won't let me do what I want!" Compositional data analysis has a pretty standard set of statistical approaches. Chemists use it all the time. These approaches are many times called "mixture designs": itl.nist.gov/div898/handbook/pri/section5/pri54.htm. Maybe you've been talking to the wrong folks.
$endgroup$
– JimB
yesterday
$begingroup$
I'm sure there must be more to it than "They won't let me do what I want!" Compositional data analysis has a pretty standard set of statistical approaches. Chemists use it all the time. These approaches are many times called "mixture designs": itl.nist.gov/div898/handbook/pri/section5/pri54.htm. Maybe you've been talking to the wrong folks.
$endgroup$
– JimB
yesterday
$begingroup$
Like I said, geologists don't. Perhaps it has to do with the general 'noisiness' of geoscience datasets. Most other sciences are able to rely on fairly precise numbers.
$endgroup$
– geordie
yesterday
$begingroup$
Like I said, geologists don't. Perhaps it has to do with the general 'noisiness' of geoscience datasets. Most other sciences are able to rely on fairly precise numbers.
$endgroup$
– geordie
yesterday
1
1
$begingroup$
"Most other sciences are able to rely on fairly precise numbers." Ha, ha. You're funny!
$endgroup$
– JimB
yesterday
$begingroup$
"Most other sciences are able to rely on fairly precise numbers." Ha, ha. You're funny!
$endgroup$
– JimB
yesterday
|
show 2 more comments
2 Answers
2
active
oldest
votes
$begingroup$
One approach is to reframe it as a minimization problem:
xVec = Array[x, 13];
NMinimize[{Total[(a.xVec - b)^2], Total[xVec] == 1, Thread[xVec >= 0]}, xVec]
You can also get an improved residual by relaxing the equality constraint:
NMinimize[{Total[(a.xVec - b)^2] + (Total[xVec] - 1)^2, Thread[xVec >= 0]}, xVec]
Thanks to Roman for some simplifications.
$endgroup$
$begingroup$
Thread[xVec > 0]
would be simpler on the third line and does the same thing. Also, you can constrain the sum by equality:NMinimize[{(a.xVec-b).(a.xVec-b), Total[xVec]==1, Thread[xVec>0]}, xVec]
. I think the square inTotal[(a.xVec - b)]^2
should be taken inside of theTotal
, not outside.
$endgroup$
– Roman
yesterday
add a comment |
$begingroup$
This can be done as a linear programming problem although the optimization is not least-squares in that case. The idea is to set up new variables, which are absolute values of discrepancies between a.x.
and b
. This can be done as below.
xvec = Array[x, Length[a[[1]]]];
dvec = Array[d, Length[a]];
lpolys = a.xvec - b;
ineqs = Flatten[{Thread[xvec >= 0], Thread[dvec - lpolys >= 0],
Thread[dvec + lpolys >= 0], Total[xvec] == 1}];
Now use NMinimize
.
{min, vals} =
NMinimize[{Total[dvec], ineqs}, Join[xvec, dvec], PrecisionGoal -> 10]
Total[xvec /. vals]
(* Out[90]= {0.627674050324, {x[1] -> 0.339337833732,
x[2] -> 0.292918812222, x[3] -> 0.195908896153,
x[4] -> 0.10491683141, x[5] -> 0.0591148361052, x[6] -> 0.,
x[7] -> 0., x[8] -> 0.00181140763364, x[9] -> 0., x[10] -> 0.,
x[11] -> 0., x[12] -> 0., x[13] -> 0.00599138274489, d[1] -> 0.,
d[2] -> 0., d[3] -> 0.502611255236, d[4] -> 0.,
d[5] -> 0.0178984583702, d[6] -> 0.0717916527728, d[7] -> 0.,
d[8] -> 0., d[9] -> 0., d[10] -> 0.0353726839451}}
Out[91]= 1. *)
$endgroup$
add a comment |
Your Answer
StackExchange.ifUsing("editor", function () {
return StackExchange.using("mathjaxEditing", function () {
StackExchange.MarkdownEditor.creationCallbacks.add(function (editor, postfix) {
StackExchange.mathjaxEditing.prepareWmdForMathJax(editor, postfix, [["$", "$"], ["\\(","\\)"]]);
});
});
}, "mathjax-editing");
StackExchange.ready(function() {
var channelOptions = {
tags: "".split(" "),
id: "387"
};
initTagRenderer("".split(" "), "".split(" "), channelOptions);
StackExchange.using("externalEditor", function() {
// Have to fire editor after snippets, if snippets enabled
if (StackExchange.settings.snippets.snippetsEnabled) {
StackExchange.using("snippets", function() {
createEditor();
});
}
else {
createEditor();
}
});
function createEditor() {
StackExchange.prepareEditor({
heartbeatType: 'answer',
autoActivateHeartbeat: false,
convertImagesToLinks: false,
noModals: true,
showLowRepImageUploadWarning: true,
reputationToPostImages: null,
bindNavPrevention: true,
postfix: "",
imageUploader: {
brandingHtml: "Powered by u003ca class="icon-imgur-white" href="https://imgur.com/"u003eu003c/au003e",
contentPolicyHtml: "User contributions licensed under u003ca href="https://creativecommons.org/licenses/by-sa/3.0/"u003ecc by-sa 3.0 with attribution requiredu003c/au003e u003ca href="https://stackoverflow.com/legal/content-policy"u003e(content policy)u003c/au003e",
allowUrls: true
},
onDemand: true,
discardSelector: ".discard-answer"
,immediatelyShowMarkdownHelp:true
});
}
});
Sign up or log in
StackExchange.ready(function () {
StackExchange.helpers.onClickDraftSave('#login-link');
});
Sign up using Google
Sign up using Facebook
Sign up using Email and Password
Post as a guest
Required, but never shown
StackExchange.ready(
function () {
StackExchange.openid.initPostLogin('.new-post-login', 'https%3a%2f%2fmathematica.stackexchange.com%2fquestions%2f193638%2fdetermining-multivariate-least-squares-with-constraint%23new-answer', 'question_page');
}
);
Post as a guest
Required, but never shown
2 Answers
2
active
oldest
votes
2 Answers
2
active
oldest
votes
active
oldest
votes
active
oldest
votes
$begingroup$
One approach is to reframe it as a minimization problem:
xVec = Array[x, 13];
NMinimize[{Total[(a.xVec - b)^2], Total[xVec] == 1, Thread[xVec >= 0]}, xVec]
You can also get an improved residual by relaxing the equality constraint:
NMinimize[{Total[(a.xVec - b)^2] + (Total[xVec] - 1)^2, Thread[xVec >= 0]}, xVec]
Thanks to Roman for some simplifications.
$endgroup$
$begingroup$
Thread[xVec > 0]
would be simpler on the third line and does the same thing. Also, you can constrain the sum by equality:NMinimize[{(a.xVec-b).(a.xVec-b), Total[xVec]==1, Thread[xVec>0]}, xVec]
. I think the square inTotal[(a.xVec - b)]^2
should be taken inside of theTotal
, not outside.
$endgroup$
– Roman
yesterday
add a comment |
$begingroup$
One approach is to reframe it as a minimization problem:
xVec = Array[x, 13];
NMinimize[{Total[(a.xVec - b)^2], Total[xVec] == 1, Thread[xVec >= 0]}, xVec]
You can also get an improved residual by relaxing the equality constraint:
NMinimize[{Total[(a.xVec - b)^2] + (Total[xVec] - 1)^2, Thread[xVec >= 0]}, xVec]
Thanks to Roman for some simplifications.
$endgroup$
$begingroup$
Thread[xVec > 0]
would be simpler on the third line and does the same thing. Also, you can constrain the sum by equality:NMinimize[{(a.xVec-b).(a.xVec-b), Total[xVec]==1, Thread[xVec>0]}, xVec]
. I think the square inTotal[(a.xVec - b)]^2
should be taken inside of theTotal
, not outside.
$endgroup$
– Roman
yesterday
add a comment |
$begingroup$
One approach is to reframe it as a minimization problem:
xVec = Array[x, 13];
NMinimize[{Total[(a.xVec - b)^2], Total[xVec] == 1, Thread[xVec >= 0]}, xVec]
You can also get an improved residual by relaxing the equality constraint:
NMinimize[{Total[(a.xVec - b)^2] + (Total[xVec] - 1)^2, Thread[xVec >= 0]}, xVec]
Thanks to Roman for some simplifications.
$endgroup$
One approach is to reframe it as a minimization problem:
xVec = Array[x, 13];
NMinimize[{Total[(a.xVec - b)^2], Total[xVec] == 1, Thread[xVec >= 0]}, xVec]
You can also get an improved residual by relaxing the equality constraint:
NMinimize[{Total[(a.xVec - b)^2] + (Total[xVec] - 1)^2, Thread[xVec >= 0]}, xVec]
Thanks to Roman for some simplifications.
edited yesterday
answered yesterday


bill sbill s
54.5k377156
54.5k377156
$begingroup$
Thread[xVec > 0]
would be simpler on the third line and does the same thing. Also, you can constrain the sum by equality:NMinimize[{(a.xVec-b).(a.xVec-b), Total[xVec]==1, Thread[xVec>0]}, xVec]
. I think the square inTotal[(a.xVec - b)]^2
should be taken inside of theTotal
, not outside.
$endgroup$
– Roman
yesterday
add a comment |
$begingroup$
Thread[xVec > 0]
would be simpler on the third line and does the same thing. Also, you can constrain the sum by equality:NMinimize[{(a.xVec-b).(a.xVec-b), Total[xVec]==1, Thread[xVec>0]}, xVec]
. I think the square inTotal[(a.xVec - b)]^2
should be taken inside of theTotal
, not outside.
$endgroup$
– Roman
yesterday
$begingroup$
Thread[xVec > 0]
would be simpler on the third line and does the same thing. Also, you can constrain the sum by equality: NMinimize[{(a.xVec-b).(a.xVec-b), Total[xVec]==1, Thread[xVec>0]}, xVec]
. I think the square in Total[(a.xVec - b)]^2
should be taken inside of the Total
, not outside.$endgroup$
– Roman
yesterday
$begingroup$
Thread[xVec > 0]
would be simpler on the third line and does the same thing. Also, you can constrain the sum by equality: NMinimize[{(a.xVec-b).(a.xVec-b), Total[xVec]==1, Thread[xVec>0]}, xVec]
. I think the square in Total[(a.xVec - b)]^2
should be taken inside of the Total
, not outside.$endgroup$
– Roman
yesterday
add a comment |
$begingroup$
This can be done as a linear programming problem although the optimization is not least-squares in that case. The idea is to set up new variables, which are absolute values of discrepancies between a.x.
and b
. This can be done as below.
xvec = Array[x, Length[a[[1]]]];
dvec = Array[d, Length[a]];
lpolys = a.xvec - b;
ineqs = Flatten[{Thread[xvec >= 0], Thread[dvec - lpolys >= 0],
Thread[dvec + lpolys >= 0], Total[xvec] == 1}];
Now use NMinimize
.
{min, vals} =
NMinimize[{Total[dvec], ineqs}, Join[xvec, dvec], PrecisionGoal -> 10]
Total[xvec /. vals]
(* Out[90]= {0.627674050324, {x[1] -> 0.339337833732,
x[2] -> 0.292918812222, x[3] -> 0.195908896153,
x[4] -> 0.10491683141, x[5] -> 0.0591148361052, x[6] -> 0.,
x[7] -> 0., x[8] -> 0.00181140763364, x[9] -> 0., x[10] -> 0.,
x[11] -> 0., x[12] -> 0., x[13] -> 0.00599138274489, d[1] -> 0.,
d[2] -> 0., d[3] -> 0.502611255236, d[4] -> 0.,
d[5] -> 0.0178984583702, d[6] -> 0.0717916527728, d[7] -> 0.,
d[8] -> 0., d[9] -> 0., d[10] -> 0.0353726839451}}
Out[91]= 1. *)
$endgroup$
add a comment |
$begingroup$
This can be done as a linear programming problem although the optimization is not least-squares in that case. The idea is to set up new variables, which are absolute values of discrepancies between a.x.
and b
. This can be done as below.
xvec = Array[x, Length[a[[1]]]];
dvec = Array[d, Length[a]];
lpolys = a.xvec - b;
ineqs = Flatten[{Thread[xvec >= 0], Thread[dvec - lpolys >= 0],
Thread[dvec + lpolys >= 0], Total[xvec] == 1}];
Now use NMinimize
.
{min, vals} =
NMinimize[{Total[dvec], ineqs}, Join[xvec, dvec], PrecisionGoal -> 10]
Total[xvec /. vals]
(* Out[90]= {0.627674050324, {x[1] -> 0.339337833732,
x[2] -> 0.292918812222, x[3] -> 0.195908896153,
x[4] -> 0.10491683141, x[5] -> 0.0591148361052, x[6] -> 0.,
x[7] -> 0., x[8] -> 0.00181140763364, x[9] -> 0., x[10] -> 0.,
x[11] -> 0., x[12] -> 0., x[13] -> 0.00599138274489, d[1] -> 0.,
d[2] -> 0., d[3] -> 0.502611255236, d[4] -> 0.,
d[5] -> 0.0178984583702, d[6] -> 0.0717916527728, d[7] -> 0.,
d[8] -> 0., d[9] -> 0., d[10] -> 0.0353726839451}}
Out[91]= 1. *)
$endgroup$
add a comment |
$begingroup$
This can be done as a linear programming problem although the optimization is not least-squares in that case. The idea is to set up new variables, which are absolute values of discrepancies between a.x.
and b
. This can be done as below.
xvec = Array[x, Length[a[[1]]]];
dvec = Array[d, Length[a]];
lpolys = a.xvec - b;
ineqs = Flatten[{Thread[xvec >= 0], Thread[dvec - lpolys >= 0],
Thread[dvec + lpolys >= 0], Total[xvec] == 1}];
Now use NMinimize
.
{min, vals} =
NMinimize[{Total[dvec], ineqs}, Join[xvec, dvec], PrecisionGoal -> 10]
Total[xvec /. vals]
(* Out[90]= {0.627674050324, {x[1] -> 0.339337833732,
x[2] -> 0.292918812222, x[3] -> 0.195908896153,
x[4] -> 0.10491683141, x[5] -> 0.0591148361052, x[6] -> 0.,
x[7] -> 0., x[8] -> 0.00181140763364, x[9] -> 0., x[10] -> 0.,
x[11] -> 0., x[12] -> 0., x[13] -> 0.00599138274489, d[1] -> 0.,
d[2] -> 0., d[3] -> 0.502611255236, d[4] -> 0.,
d[5] -> 0.0178984583702, d[6] -> 0.0717916527728, d[7] -> 0.,
d[8] -> 0., d[9] -> 0., d[10] -> 0.0353726839451}}
Out[91]= 1. *)
$endgroup$
This can be done as a linear programming problem although the optimization is not least-squares in that case. The idea is to set up new variables, which are absolute values of discrepancies between a.x.
and b
. This can be done as below.
xvec = Array[x, Length[a[[1]]]];
dvec = Array[d, Length[a]];
lpolys = a.xvec - b;
ineqs = Flatten[{Thread[xvec >= 0], Thread[dvec - lpolys >= 0],
Thread[dvec + lpolys >= 0], Total[xvec] == 1}];
Now use NMinimize
.
{min, vals} =
NMinimize[{Total[dvec], ineqs}, Join[xvec, dvec], PrecisionGoal -> 10]
Total[xvec /. vals]
(* Out[90]= {0.627674050324, {x[1] -> 0.339337833732,
x[2] -> 0.292918812222, x[3] -> 0.195908896153,
x[4] -> 0.10491683141, x[5] -> 0.0591148361052, x[6] -> 0.,
x[7] -> 0., x[8] -> 0.00181140763364, x[9] -> 0., x[10] -> 0.,
x[11] -> 0., x[12] -> 0., x[13] -> 0.00599138274489, d[1] -> 0.,
d[2] -> 0., d[3] -> 0.502611255236, d[4] -> 0.,
d[5] -> 0.0178984583702, d[6] -> 0.0717916527728, d[7] -> 0.,
d[8] -> 0., d[9] -> 0., d[10] -> 0.0353726839451}}
Out[91]= 1. *)
answered yesterday
Daniel LichtblauDaniel Lichtblau
47.3k276164
47.3k276164
add a comment |
add a comment |
Thanks for contributing an answer to Mathematica Stack Exchange!
- Please be sure to answer the question. Provide details and share your research!
But avoid …
- Asking for help, clarification, or responding to other answers.
- Making statements based on opinion; back them up with references or personal experience.
Use MathJax to format equations. MathJax reference.
To learn more, see our tips on writing great answers.
Sign up or log in
StackExchange.ready(function () {
StackExchange.helpers.onClickDraftSave('#login-link');
});
Sign up using Google
Sign up using Facebook
Sign up using Email and Password
Post as a guest
Required, but never shown
StackExchange.ready(
function () {
StackExchange.openid.initPostLogin('.new-post-login', 'https%3a%2f%2fmathematica.stackexchange.com%2fquestions%2f193638%2fdetermining-multivariate-least-squares-with-constraint%23new-answer', 'question_page');
}
);
Post as a guest
Required, but never shown
Sign up or log in
StackExchange.ready(function () {
StackExchange.helpers.onClickDraftSave('#login-link');
});
Sign up using Google
Sign up using Facebook
Sign up using Email and Password
Post as a guest
Required, but never shown
Sign up or log in
StackExchange.ready(function () {
StackExchange.helpers.onClickDraftSave('#login-link');
});
Sign up using Google
Sign up using Facebook
Sign up using Email and Password
Post as a guest
Required, but never shown
Sign up or log in
StackExchange.ready(function () {
StackExchange.helpers.onClickDraftSave('#login-link');
});
Sign up using Google
Sign up using Facebook
Sign up using Email and Password
Sign up using Google
Sign up using Facebook
Sign up using Email and Password
Post as a guest
Required, but never shown
Required, but never shown
Required, but never shown
Required, but never shown
Required, but never shown
Required, but never shown
Required, but never shown
Required, but never shown
Required, but never shown
iKoAYLoJ,YS I2LvMtGdg U ZdbXlc0E,5XJZw fM mQqcGaENinrZwyIJa7EZSvz bUTXXs,DIGj2tM5FN
2
$begingroup$
My usual soapbox speech: Why not consult a statistician first and then figure out how to implement an appropriate process in Mathematica? Consider stats.stackexchange.com/questions/267014/….
$endgroup$
– JimB
yesterday
$begingroup$
@JimB precisely because someone will tell me that I can't use continuum methods with compositional data. The constant sum problem is considered a vague oddity in geological circles and largely ignored (for better or worse).
$endgroup$
– geordie
yesterday
$begingroup$
I'm sure there must be more to it than "They won't let me do what I want!" Compositional data analysis has a pretty standard set of statistical approaches. Chemists use it all the time. These approaches are many times called "mixture designs": itl.nist.gov/div898/handbook/pri/section5/pri54.htm. Maybe you've been talking to the wrong folks.
$endgroup$
– JimB
yesterday
$begingroup$
Like I said, geologists don't. Perhaps it has to do with the general 'noisiness' of geoscience datasets. Most other sciences are able to rely on fairly precise numbers.
$endgroup$
– geordie
yesterday
1
$begingroup$
"Most other sciences are able to rely on fairly precise numbers." Ha, ha. You're funny!
$endgroup$
– JimB
yesterday