Justifying the trend component in a time series?
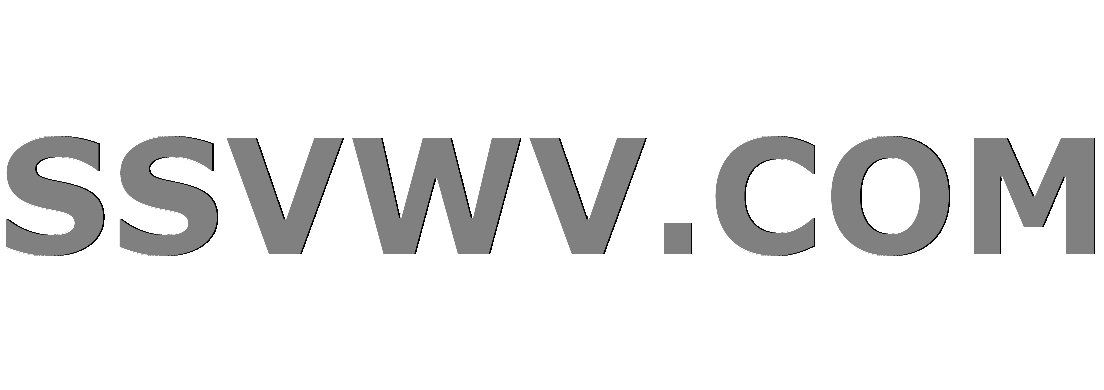
Multi tool use
$begingroup$
I am working on a time series forecasting problem ,I used Dickey–Fuller test to check the stationary of the time series and the p value is 0.000835 , hense I rejected the null hypothesis and assumed that it's a stationary time series.
I decomposed the time series and the trend component does not have any particular pattern.plot-
I want to ask how can we justify that a time series has any particular trend, Is this decomposition enough to ensure and guarantee that this time series has no trend ?
time-series statistical-significance
New contributor
A.kumar is a new contributor to this site. Take care in asking for clarification, commenting, and answering.
Check out our Code of Conduct.
$endgroup$
add a comment |
$begingroup$
I am working on a time series forecasting problem ,I used Dickey–Fuller test to check the stationary of the time series and the p value is 0.000835 , hense I rejected the null hypothesis and assumed that it's a stationary time series.
I decomposed the time series and the trend component does not have any particular pattern.plot-
I want to ask how can we justify that a time series has any particular trend, Is this decomposition enough to ensure and guarantee that this time series has no trend ?
time-series statistical-significance
New contributor
A.kumar is a new contributor to this site. Take care in asking for clarification, commenting, and answering.
Check out our Code of Conduct.
$endgroup$
add a comment |
$begingroup$
I am working on a time series forecasting problem ,I used Dickey–Fuller test to check the stationary of the time series and the p value is 0.000835 , hense I rejected the null hypothesis and assumed that it's a stationary time series.
I decomposed the time series and the trend component does not have any particular pattern.plot-
I want to ask how can we justify that a time series has any particular trend, Is this decomposition enough to ensure and guarantee that this time series has no trend ?
time-series statistical-significance
New contributor
A.kumar is a new contributor to this site. Take care in asking for clarification, commenting, and answering.
Check out our Code of Conduct.
$endgroup$
I am working on a time series forecasting problem ,I used Dickey–Fuller test to check the stationary of the time series and the p value is 0.000835 , hense I rejected the null hypothesis and assumed that it's a stationary time series.
I decomposed the time series and the trend component does not have any particular pattern.plot-
I want to ask how can we justify that a time series has any particular trend, Is this decomposition enough to ensure and guarantee that this time series has no trend ?
time-series statistical-significance
time-series statistical-significance
New contributor
A.kumar is a new contributor to this site. Take care in asking for clarification, commenting, and answering.
Check out our Code of Conduct.
New contributor
A.kumar is a new contributor to this site. Take care in asking for clarification, commenting, and answering.
Check out our Code of Conduct.
New contributor
A.kumar is a new contributor to this site. Take care in asking for clarification, commenting, and answering.
Check out our Code of Conduct.
asked 16 hours ago
A.kumarA.kumar
111
111
New contributor
A.kumar is a new contributor to this site. Take care in asking for clarification, commenting, and answering.
Check out our Code of Conduct.
New contributor
A.kumar is a new contributor to this site. Take care in asking for clarification, commenting, and answering.
Check out our Code of Conduct.
A.kumar is a new contributor to this site. Take care in asking for clarification, commenting, and answering.
Check out our Code of Conduct.
add a comment |
add a comment |
2 Answers
2
active
oldest
votes
$begingroup$
The Augmented Dickey–Fuller test is UNIT ROOT test - NOT a stationarity test. The null-hypothesis on this test is that the data have been generated by a restricted AR model containing a unit-root. Rejecting this hypothesis DOES NOT mean the series is stationary. It just means that there is enough evidence to allow you rejecting the hypothesis of a very specific form of NON-stationarity (i.e. unit root). Evidence is assessed contrastively i.e. v.s. an unrestricted AR model. Rejecting the hypothesis that an animal is a chicken with an alternative being a pig doesn't mean the animal might not be a horse! The examined animal might contrastively not look like a chicken (e.g. because it has 4 legs) but that doesn't make it a pig.
Now look at your decomposition. You could see with a bare eye data don't look stationary. Trend as well as variance change with time. The decomposition does not support your conclusion. It supports the opposite view. There both parametric as well as non-parametric ways to check changes in the trend of the series. For example (see following poster about Detecting Changes in the Mean ):
$endgroup$
add a comment |
$begingroup$
visually there is an apparent autoregressive process and a change in model error variance .. thus non-stationarity is the call.
$endgroup$
add a comment |
Your Answer
StackExchange.ifUsing("editor", function () {
return StackExchange.using("mathjaxEditing", function () {
StackExchange.MarkdownEditor.creationCallbacks.add(function (editor, postfix) {
StackExchange.mathjaxEditing.prepareWmdForMathJax(editor, postfix, [["$", "$"], ["\\(","\\)"]]);
});
});
}, "mathjax-editing");
StackExchange.ready(function() {
var channelOptions = {
tags: "".split(" "),
id: "65"
};
initTagRenderer("".split(" "), "".split(" "), channelOptions);
StackExchange.using("externalEditor", function() {
// Have to fire editor after snippets, if snippets enabled
if (StackExchange.settings.snippets.snippetsEnabled) {
StackExchange.using("snippets", function() {
createEditor();
});
}
else {
createEditor();
}
});
function createEditor() {
StackExchange.prepareEditor({
heartbeatType: 'answer',
autoActivateHeartbeat: false,
convertImagesToLinks: false,
noModals: true,
showLowRepImageUploadWarning: true,
reputationToPostImages: null,
bindNavPrevention: true,
postfix: "",
imageUploader: {
brandingHtml: "Powered by u003ca class="icon-imgur-white" href="https://imgur.com/"u003eu003c/au003e",
contentPolicyHtml: "User contributions licensed under u003ca href="https://creativecommons.org/licenses/by-sa/3.0/"u003ecc by-sa 3.0 with attribution requiredu003c/au003e u003ca href="https://stackoverflow.com/legal/content-policy"u003e(content policy)u003c/au003e",
allowUrls: true
},
onDemand: true,
discardSelector: ".discard-answer"
,immediatelyShowMarkdownHelp:true
});
}
});
A.kumar is a new contributor. Be nice, and check out our Code of Conduct.
Sign up or log in
StackExchange.ready(function () {
StackExchange.helpers.onClickDraftSave('#login-link');
});
Sign up using Google
Sign up using Facebook
Sign up using Email and Password
Post as a guest
Required, but never shown
StackExchange.ready(
function () {
StackExchange.openid.initPostLogin('.new-post-login', 'https%3a%2f%2fstats.stackexchange.com%2fquestions%2f397464%2fjustifying-the-trend-component-in-a-time-series%23new-answer', 'question_page');
}
);
Post as a guest
Required, but never shown
2 Answers
2
active
oldest
votes
2 Answers
2
active
oldest
votes
active
oldest
votes
active
oldest
votes
$begingroup$
The Augmented Dickey–Fuller test is UNIT ROOT test - NOT a stationarity test. The null-hypothesis on this test is that the data have been generated by a restricted AR model containing a unit-root. Rejecting this hypothesis DOES NOT mean the series is stationary. It just means that there is enough evidence to allow you rejecting the hypothesis of a very specific form of NON-stationarity (i.e. unit root). Evidence is assessed contrastively i.e. v.s. an unrestricted AR model. Rejecting the hypothesis that an animal is a chicken with an alternative being a pig doesn't mean the animal might not be a horse! The examined animal might contrastively not look like a chicken (e.g. because it has 4 legs) but that doesn't make it a pig.
Now look at your decomposition. You could see with a bare eye data don't look stationary. Trend as well as variance change with time. The decomposition does not support your conclusion. It supports the opposite view. There both parametric as well as non-parametric ways to check changes in the trend of the series. For example (see following poster about Detecting Changes in the Mean ):
$endgroup$
add a comment |
$begingroup$
The Augmented Dickey–Fuller test is UNIT ROOT test - NOT a stationarity test. The null-hypothesis on this test is that the data have been generated by a restricted AR model containing a unit-root. Rejecting this hypothesis DOES NOT mean the series is stationary. It just means that there is enough evidence to allow you rejecting the hypothesis of a very specific form of NON-stationarity (i.e. unit root). Evidence is assessed contrastively i.e. v.s. an unrestricted AR model. Rejecting the hypothesis that an animal is a chicken with an alternative being a pig doesn't mean the animal might not be a horse! The examined animal might contrastively not look like a chicken (e.g. because it has 4 legs) but that doesn't make it a pig.
Now look at your decomposition. You could see with a bare eye data don't look stationary. Trend as well as variance change with time. The decomposition does not support your conclusion. It supports the opposite view. There both parametric as well as non-parametric ways to check changes in the trend of the series. For example (see following poster about Detecting Changes in the Mean ):
$endgroup$
add a comment |
$begingroup$
The Augmented Dickey–Fuller test is UNIT ROOT test - NOT a stationarity test. The null-hypothesis on this test is that the data have been generated by a restricted AR model containing a unit-root. Rejecting this hypothesis DOES NOT mean the series is stationary. It just means that there is enough evidence to allow you rejecting the hypothesis of a very specific form of NON-stationarity (i.e. unit root). Evidence is assessed contrastively i.e. v.s. an unrestricted AR model. Rejecting the hypothesis that an animal is a chicken with an alternative being a pig doesn't mean the animal might not be a horse! The examined animal might contrastively not look like a chicken (e.g. because it has 4 legs) but that doesn't make it a pig.
Now look at your decomposition. You could see with a bare eye data don't look stationary. Trend as well as variance change with time. The decomposition does not support your conclusion. It supports the opposite view. There both parametric as well as non-parametric ways to check changes in the trend of the series. For example (see following poster about Detecting Changes in the Mean ):
$endgroup$
The Augmented Dickey–Fuller test is UNIT ROOT test - NOT a stationarity test. The null-hypothesis on this test is that the data have been generated by a restricted AR model containing a unit-root. Rejecting this hypothesis DOES NOT mean the series is stationary. It just means that there is enough evidence to allow you rejecting the hypothesis of a very specific form of NON-stationarity (i.e. unit root). Evidence is assessed contrastively i.e. v.s. an unrestricted AR model. Rejecting the hypothesis that an animal is a chicken with an alternative being a pig doesn't mean the animal might not be a horse! The examined animal might contrastively not look like a chicken (e.g. because it has 4 legs) but that doesn't make it a pig.
Now look at your decomposition. You could see with a bare eye data don't look stationary. Trend as well as variance change with time. The decomposition does not support your conclusion. It supports the opposite view. There both parametric as well as non-parametric ways to check changes in the trend of the series. For example (see following poster about Detecting Changes in the Mean ):
edited 13 hours ago
answered 13 hours ago
StatsStats
50619
50619
add a comment |
add a comment |
$begingroup$
visually there is an apparent autoregressive process and a change in model error variance .. thus non-stationarity is the call.
$endgroup$
add a comment |
$begingroup$
visually there is an apparent autoregressive process and a change in model error variance .. thus non-stationarity is the call.
$endgroup$
add a comment |
$begingroup$
visually there is an apparent autoregressive process and a change in model error variance .. thus non-stationarity is the call.
$endgroup$
visually there is an apparent autoregressive process and a change in model error variance .. thus non-stationarity is the call.
answered 13 hours ago


IrishStatIrishStat
21k42241
21k42241
add a comment |
add a comment |
A.kumar is a new contributor. Be nice, and check out our Code of Conduct.
A.kumar is a new contributor. Be nice, and check out our Code of Conduct.
A.kumar is a new contributor. Be nice, and check out our Code of Conduct.
A.kumar is a new contributor. Be nice, and check out our Code of Conduct.
Thanks for contributing an answer to Cross Validated!
- Please be sure to answer the question. Provide details and share your research!
But avoid …
- Asking for help, clarification, or responding to other answers.
- Making statements based on opinion; back them up with references or personal experience.
Use MathJax to format equations. MathJax reference.
To learn more, see our tips on writing great answers.
Sign up or log in
StackExchange.ready(function () {
StackExchange.helpers.onClickDraftSave('#login-link');
});
Sign up using Google
Sign up using Facebook
Sign up using Email and Password
Post as a guest
Required, but never shown
StackExchange.ready(
function () {
StackExchange.openid.initPostLogin('.new-post-login', 'https%3a%2f%2fstats.stackexchange.com%2fquestions%2f397464%2fjustifying-the-trend-component-in-a-time-series%23new-answer', 'question_page');
}
);
Post as a guest
Required, but never shown
Sign up or log in
StackExchange.ready(function () {
StackExchange.helpers.onClickDraftSave('#login-link');
});
Sign up using Google
Sign up using Facebook
Sign up using Email and Password
Post as a guest
Required, but never shown
Sign up or log in
StackExchange.ready(function () {
StackExchange.helpers.onClickDraftSave('#login-link');
});
Sign up using Google
Sign up using Facebook
Sign up using Email and Password
Post as a guest
Required, but never shown
Sign up or log in
StackExchange.ready(function () {
StackExchange.helpers.onClickDraftSave('#login-link');
});
Sign up using Google
Sign up using Facebook
Sign up using Email and Password
Sign up using Google
Sign up using Facebook
Sign up using Email and Password
Post as a guest
Required, but never shown
Required, but never shown
Required, but never shown
Required, but never shown
Required, but never shown
Required, but never shown
Required, but never shown
Required, but never shown
Required, but never shown
cDQOM gfE 35RSdd37kkT7