Understanding minimizing cost correctly
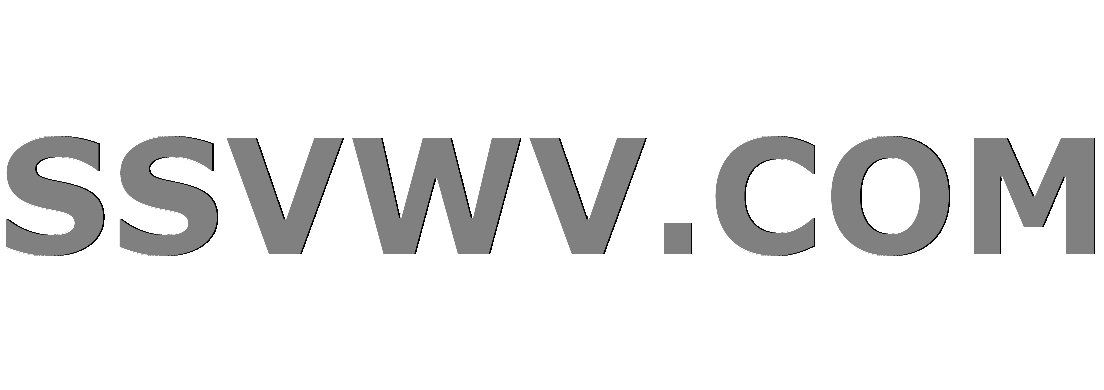
Multi tool use
$begingroup$
I cannot wrap my head around this simple concept.
Suppose we have a linear regression, and there is a single parameter theta to be optimized (for simplicity purposes):
$h(x) = theta cdot x$
The error cost function could be defined as $J(theta) = frac1m cdot sum (h(x) - y(x)) ^ 2$, for each $x$.
Then, theta would be updated as:
$theta = theta - alphacdot frac1m cdot sum (h(x) - y(x)) cdot x$, for each $x$.
From my understanding the multiplier after the alpha term is the derivative of the error cost function $J$. This term tells us the direction to head in, in order to arrive at the minimum making a small step at a time. I understand the concept of "hill climbing" correctly, at least I think.
Here is where I don't seem to wrap my head around:
If the form of the error function is known (like in our case: we could visually plot the function if we take enough values of theta and plug them in the model), why can't we take the first derivative and set it to zero (partial derivative if the function has multiple thetas). This way we would have all the minimums of the function. Then with the second derivative, we could determine whether it's a min or a max.
I've seen this done in calculus for simple functions like $y = x^2 + 5x + 2$ (may years ago, maybe I am wrong), so what is stopping us from doing the same thing here?
Sorry for asking such a silly question.
Thank you.
linear-regression cost-function
$endgroup$
add a comment |
$begingroup$
I cannot wrap my head around this simple concept.
Suppose we have a linear regression, and there is a single parameter theta to be optimized (for simplicity purposes):
$h(x) = theta cdot x$
The error cost function could be defined as $J(theta) = frac1m cdot sum (h(x) - y(x)) ^ 2$, for each $x$.
Then, theta would be updated as:
$theta = theta - alphacdot frac1m cdot sum (h(x) - y(x)) cdot x$, for each $x$.
From my understanding the multiplier after the alpha term is the derivative of the error cost function $J$. This term tells us the direction to head in, in order to arrive at the minimum making a small step at a time. I understand the concept of "hill climbing" correctly, at least I think.
Here is where I don't seem to wrap my head around:
If the form of the error function is known (like in our case: we could visually plot the function if we take enough values of theta and plug them in the model), why can't we take the first derivative and set it to zero (partial derivative if the function has multiple thetas). This way we would have all the minimums of the function. Then with the second derivative, we could determine whether it's a min or a max.
I've seen this done in calculus for simple functions like $y = x^2 + 5x + 2$ (may years ago, maybe I am wrong), so what is stopping us from doing the same thing here?
Sorry for asking such a silly question.
Thank you.
linear-regression cost-function
$endgroup$
add a comment |
$begingroup$
I cannot wrap my head around this simple concept.
Suppose we have a linear regression, and there is a single parameter theta to be optimized (for simplicity purposes):
$h(x) = theta cdot x$
The error cost function could be defined as $J(theta) = frac1m cdot sum (h(x) - y(x)) ^ 2$, for each $x$.
Then, theta would be updated as:
$theta = theta - alphacdot frac1m cdot sum (h(x) - y(x)) cdot x$, for each $x$.
From my understanding the multiplier after the alpha term is the derivative of the error cost function $J$. This term tells us the direction to head in, in order to arrive at the minimum making a small step at a time. I understand the concept of "hill climbing" correctly, at least I think.
Here is where I don't seem to wrap my head around:
If the form of the error function is known (like in our case: we could visually plot the function if we take enough values of theta and plug them in the model), why can't we take the first derivative and set it to zero (partial derivative if the function has multiple thetas). This way we would have all the minimums of the function. Then with the second derivative, we could determine whether it's a min or a max.
I've seen this done in calculus for simple functions like $y = x^2 + 5x + 2$ (may years ago, maybe I am wrong), so what is stopping us from doing the same thing here?
Sorry for asking such a silly question.
Thank you.
linear-regression cost-function
$endgroup$
I cannot wrap my head around this simple concept.
Suppose we have a linear regression, and there is a single parameter theta to be optimized (for simplicity purposes):
$h(x) = theta cdot x$
The error cost function could be defined as $J(theta) = frac1m cdot sum (h(x) - y(x)) ^ 2$, for each $x$.
Then, theta would be updated as:
$theta = theta - alphacdot frac1m cdot sum (h(x) - y(x)) cdot x$, for each $x$.
From my understanding the multiplier after the alpha term is the derivative of the error cost function $J$. This term tells us the direction to head in, in order to arrive at the minimum making a small step at a time. I understand the concept of "hill climbing" correctly, at least I think.
Here is where I don't seem to wrap my head around:
If the form of the error function is known (like in our case: we could visually plot the function if we take enough values of theta and plug them in the model), why can't we take the first derivative and set it to zero (partial derivative if the function has multiple thetas). This way we would have all the minimums of the function. Then with the second derivative, we could determine whether it's a min or a max.
I've seen this done in calculus for simple functions like $y = x^2 + 5x + 2$ (may years ago, maybe I am wrong), so what is stopping us from doing the same thing here?
Sorry for asking such a silly question.
Thank you.
linear-regression cost-function
linear-regression cost-function
edited Mar 17 at 10:51


Siong Thye Goh
1,387520
1,387520
asked Mar 17 at 10:33
zafirzaryazafirzarya
182
182
add a comment |
add a comment |
1 Answer
1
active
oldest
votes
$begingroup$
Consider differentiating this $$nabla_theta|Xtheta -y|^2=2X^T(Xtheta -y)=0$$
Hence solving this, would give us $$X^TXtheta =X^Ty$$
Solving this would give us the optimal solution theoretically. However, numerical stability is an issue and also don't forget computational complexity. The complexity to solve a linear system is cubic.
Also, sometimes, we do not even know even have a closed form, a gradient based approach can be more applicable.
$endgroup$
1
$begingroup$
Thank you for replying. However, I am not that mathematically literate to understand your answer. Is there a simpler answer?
$endgroup$
– zafirzarya
Mar 17 at 10:53
$begingroup$
I found an answer in MSE to illustrate why computing $X^TX$ is bad. Most approaches that aim at directly solving the normal equation is more expensive than a gradient based approach. Also such gradient based approach have been adapted to a sampling based approach as well known as stochastic gradient descent that can handle very big data.
$endgroup$
– Siong Thye Goh
Mar 17 at 11:15
add a comment |
Your Answer
StackExchange.ifUsing("editor", function () {
return StackExchange.using("mathjaxEditing", function () {
StackExchange.MarkdownEditor.creationCallbacks.add(function (editor, postfix) {
StackExchange.mathjaxEditing.prepareWmdForMathJax(editor, postfix, [["$", "$"], ["\\(","\\)"]]);
});
});
}, "mathjax-editing");
StackExchange.ready(function() {
var channelOptions = {
tags: "".split(" "),
id: "557"
};
initTagRenderer("".split(" "), "".split(" "), channelOptions);
StackExchange.using("externalEditor", function() {
// Have to fire editor after snippets, if snippets enabled
if (StackExchange.settings.snippets.snippetsEnabled) {
StackExchange.using("snippets", function() {
createEditor();
});
}
else {
createEditor();
}
});
function createEditor() {
StackExchange.prepareEditor({
heartbeatType: 'answer',
autoActivateHeartbeat: false,
convertImagesToLinks: false,
noModals: true,
showLowRepImageUploadWarning: true,
reputationToPostImages: null,
bindNavPrevention: true,
postfix: "",
imageUploader: {
brandingHtml: "Powered by u003ca class="icon-imgur-white" href="https://imgur.com/"u003eu003c/au003e",
contentPolicyHtml: "User contributions licensed under u003ca href="https://creativecommons.org/licenses/by-sa/3.0/"u003ecc by-sa 3.0 with attribution requiredu003c/au003e u003ca href="https://stackoverflow.com/legal/content-policy"u003e(content policy)u003c/au003e",
allowUrls: true
},
onDemand: true,
discardSelector: ".discard-answer"
,immediatelyShowMarkdownHelp:true
});
}
});
Sign up or log in
StackExchange.ready(function () {
StackExchange.helpers.onClickDraftSave('#login-link');
});
Sign up using Google
Sign up using Facebook
Sign up using Email and Password
Post as a guest
Required, but never shown
StackExchange.ready(
function () {
StackExchange.openid.initPostLogin('.new-post-login', 'https%3a%2f%2fdatascience.stackexchange.com%2fquestions%2f47466%2funderstanding-minimizing-cost-correctly%23new-answer', 'question_page');
}
);
Post as a guest
Required, but never shown
1 Answer
1
active
oldest
votes
1 Answer
1
active
oldest
votes
active
oldest
votes
active
oldest
votes
$begingroup$
Consider differentiating this $$nabla_theta|Xtheta -y|^2=2X^T(Xtheta -y)=0$$
Hence solving this, would give us $$X^TXtheta =X^Ty$$
Solving this would give us the optimal solution theoretically. However, numerical stability is an issue and also don't forget computational complexity. The complexity to solve a linear system is cubic.
Also, sometimes, we do not even know even have a closed form, a gradient based approach can be more applicable.
$endgroup$
1
$begingroup$
Thank you for replying. However, I am not that mathematically literate to understand your answer. Is there a simpler answer?
$endgroup$
– zafirzarya
Mar 17 at 10:53
$begingroup$
I found an answer in MSE to illustrate why computing $X^TX$ is bad. Most approaches that aim at directly solving the normal equation is more expensive than a gradient based approach. Also such gradient based approach have been adapted to a sampling based approach as well known as stochastic gradient descent that can handle very big data.
$endgroup$
– Siong Thye Goh
Mar 17 at 11:15
add a comment |
$begingroup$
Consider differentiating this $$nabla_theta|Xtheta -y|^2=2X^T(Xtheta -y)=0$$
Hence solving this, would give us $$X^TXtheta =X^Ty$$
Solving this would give us the optimal solution theoretically. However, numerical stability is an issue and also don't forget computational complexity. The complexity to solve a linear system is cubic.
Also, sometimes, we do not even know even have a closed form, a gradient based approach can be more applicable.
$endgroup$
1
$begingroup$
Thank you for replying. However, I am not that mathematically literate to understand your answer. Is there a simpler answer?
$endgroup$
– zafirzarya
Mar 17 at 10:53
$begingroup$
I found an answer in MSE to illustrate why computing $X^TX$ is bad. Most approaches that aim at directly solving the normal equation is more expensive than a gradient based approach. Also such gradient based approach have been adapted to a sampling based approach as well known as stochastic gradient descent that can handle very big data.
$endgroup$
– Siong Thye Goh
Mar 17 at 11:15
add a comment |
$begingroup$
Consider differentiating this $$nabla_theta|Xtheta -y|^2=2X^T(Xtheta -y)=0$$
Hence solving this, would give us $$X^TXtheta =X^Ty$$
Solving this would give us the optimal solution theoretically. However, numerical stability is an issue and also don't forget computational complexity. The complexity to solve a linear system is cubic.
Also, sometimes, we do not even know even have a closed form, a gradient based approach can be more applicable.
$endgroup$
Consider differentiating this $$nabla_theta|Xtheta -y|^2=2X^T(Xtheta -y)=0$$
Hence solving this, would give us $$X^TXtheta =X^Ty$$
Solving this would give us the optimal solution theoretically. However, numerical stability is an issue and also don't forget computational complexity. The complexity to solve a linear system is cubic.
Also, sometimes, we do not even know even have a closed form, a gradient based approach can be more applicable.
answered Mar 17 at 10:50


Siong Thye GohSiong Thye Goh
1,387520
1,387520
1
$begingroup$
Thank you for replying. However, I am not that mathematically literate to understand your answer. Is there a simpler answer?
$endgroup$
– zafirzarya
Mar 17 at 10:53
$begingroup$
I found an answer in MSE to illustrate why computing $X^TX$ is bad. Most approaches that aim at directly solving the normal equation is more expensive than a gradient based approach. Also such gradient based approach have been adapted to a sampling based approach as well known as stochastic gradient descent that can handle very big data.
$endgroup$
– Siong Thye Goh
Mar 17 at 11:15
add a comment |
1
$begingroup$
Thank you for replying. However, I am not that mathematically literate to understand your answer. Is there a simpler answer?
$endgroup$
– zafirzarya
Mar 17 at 10:53
$begingroup$
I found an answer in MSE to illustrate why computing $X^TX$ is bad. Most approaches that aim at directly solving the normal equation is more expensive than a gradient based approach. Also such gradient based approach have been adapted to a sampling based approach as well known as stochastic gradient descent that can handle very big data.
$endgroup$
– Siong Thye Goh
Mar 17 at 11:15
1
1
$begingroup$
Thank you for replying. However, I am not that mathematically literate to understand your answer. Is there a simpler answer?
$endgroup$
– zafirzarya
Mar 17 at 10:53
$begingroup$
Thank you for replying. However, I am not that mathematically literate to understand your answer. Is there a simpler answer?
$endgroup$
– zafirzarya
Mar 17 at 10:53
$begingroup$
I found an answer in MSE to illustrate why computing $X^TX$ is bad. Most approaches that aim at directly solving the normal equation is more expensive than a gradient based approach. Also such gradient based approach have been adapted to a sampling based approach as well known as stochastic gradient descent that can handle very big data.
$endgroup$
– Siong Thye Goh
Mar 17 at 11:15
$begingroup$
I found an answer in MSE to illustrate why computing $X^TX$ is bad. Most approaches that aim at directly solving the normal equation is more expensive than a gradient based approach. Also such gradient based approach have been adapted to a sampling based approach as well known as stochastic gradient descent that can handle very big data.
$endgroup$
– Siong Thye Goh
Mar 17 at 11:15
add a comment |
Thanks for contributing an answer to Data Science Stack Exchange!
- Please be sure to answer the question. Provide details and share your research!
But avoid …
- Asking for help, clarification, or responding to other answers.
- Making statements based on opinion; back them up with references or personal experience.
Use MathJax to format equations. MathJax reference.
To learn more, see our tips on writing great answers.
Sign up or log in
StackExchange.ready(function () {
StackExchange.helpers.onClickDraftSave('#login-link');
});
Sign up using Google
Sign up using Facebook
Sign up using Email and Password
Post as a guest
Required, but never shown
StackExchange.ready(
function () {
StackExchange.openid.initPostLogin('.new-post-login', 'https%3a%2f%2fdatascience.stackexchange.com%2fquestions%2f47466%2funderstanding-minimizing-cost-correctly%23new-answer', 'question_page');
}
);
Post as a guest
Required, but never shown
Sign up or log in
StackExchange.ready(function () {
StackExchange.helpers.onClickDraftSave('#login-link');
});
Sign up using Google
Sign up using Facebook
Sign up using Email and Password
Post as a guest
Required, but never shown
Sign up or log in
StackExchange.ready(function () {
StackExchange.helpers.onClickDraftSave('#login-link');
});
Sign up using Google
Sign up using Facebook
Sign up using Email and Password
Post as a guest
Required, but never shown
Sign up or log in
StackExchange.ready(function () {
StackExchange.helpers.onClickDraftSave('#login-link');
});
Sign up using Google
Sign up using Facebook
Sign up using Email and Password
Sign up using Google
Sign up using Facebook
Sign up using Email and Password
Post as a guest
Required, but never shown
Required, but never shown
Required, but never shown
Required, but never shown
Required, but never shown
Required, but never shown
Required, but never shown
Required, but never shown
Required, but never shown
yR7zra UT m IYcE5cf60kUuqZSuJu